25 Best Secret AI Prompts for Data Cleaning You Need to Know Today!
25 Best Secret AI Prompts for Data Cleaning You Need to Know Today!
Riley Walz
Riley Walz
Riley Walz
Feb 16, 2025
Feb 16, 2025
Feb 16, 2025
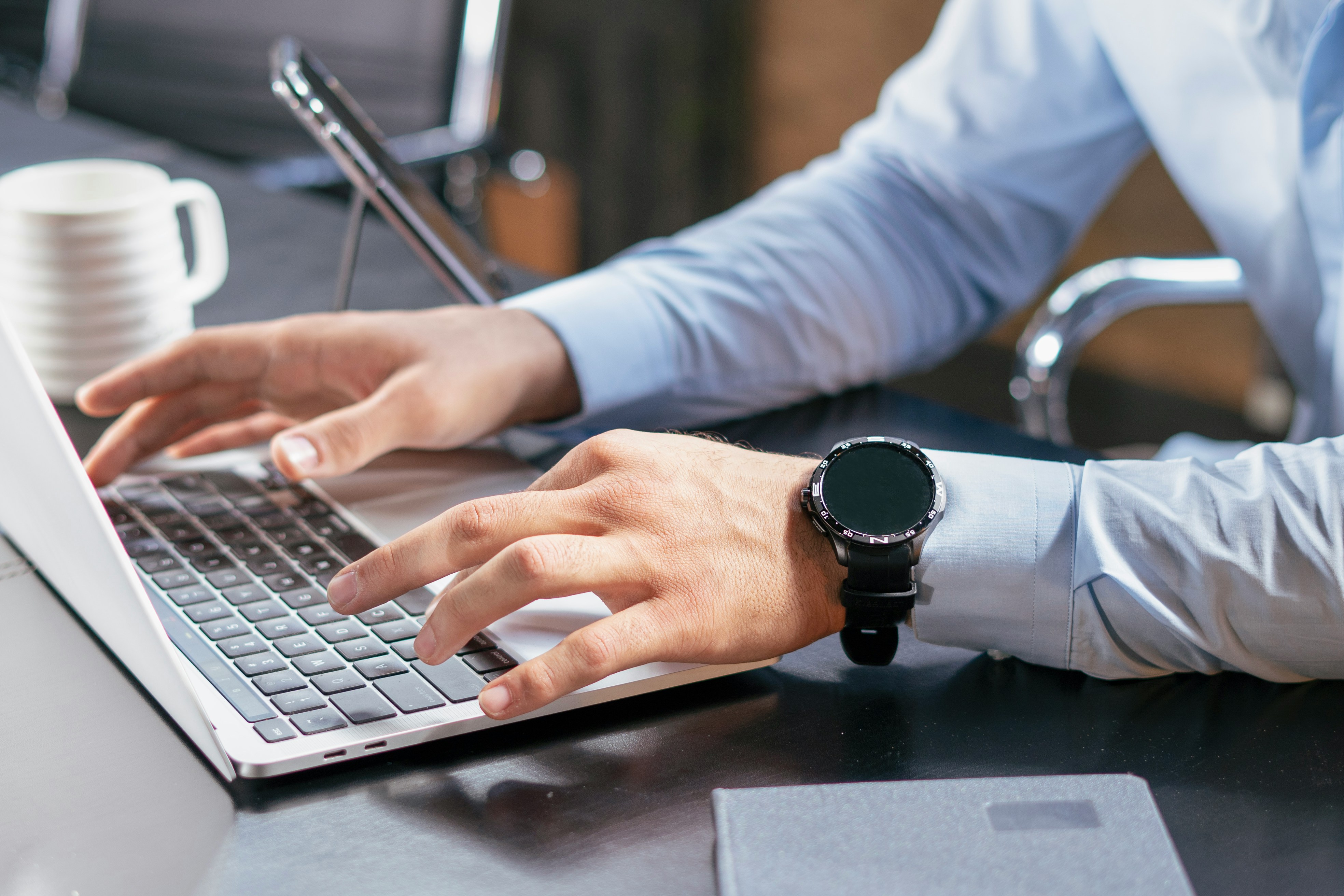
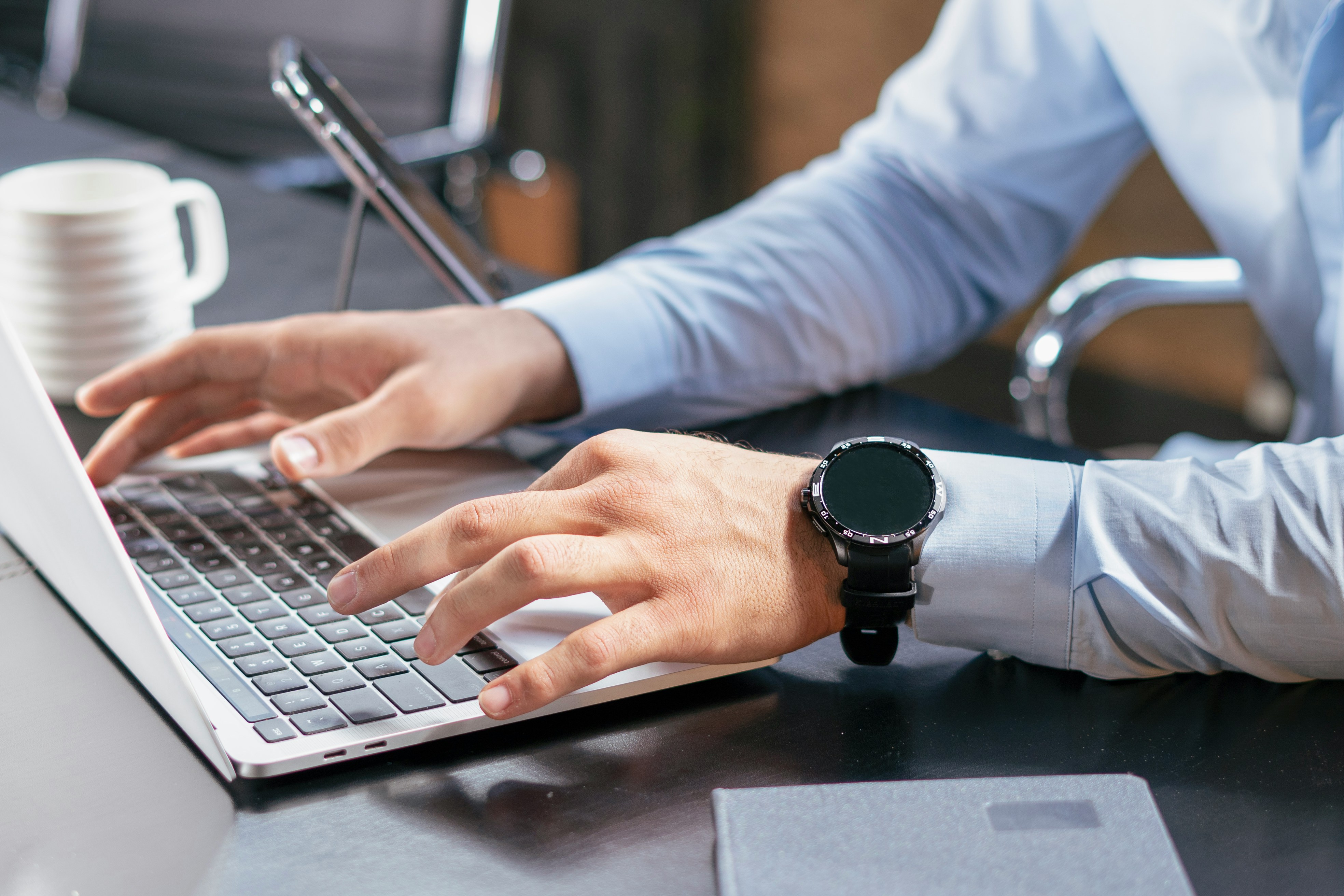
Cleaning data is like cleaning out your garage. At first, it seems like a simple task. But once you start, you realize it's much more complex than you thought. Before long, you uncover forgotten junk and hidden treasures that must be tossed, repaired, or organized. In the case of data, these items are errors and anomalies, some of which can be fixed with a simple delete or find and replace command, while others demand more intricate attention.
And just like there’s no telling how long a garage cleaning might take, the same goes for data cleaning techniques. You can't predict how long it will take until you get into the task and figure out what’s happening. The good news is that you can speed up the process and make it less painful with the right tools. This blog will introduce you to 25 of the best secret AI prompts for data cleaning you need to know today to help you uncover and organize your data’s hidden treasures.
Numerous spreadsheet AI tool is among the best for learning and implementing these prompts. It uses open AI technology to help you quickly create data-cleaning tasks and automate the process to get to your clean data faster.
Table of Content
What is Data Cleaning? Why It’s Essential for Accurate Analysis
How to Write AI Prompts for Data Cleaning (Step-by-Step Guide)
Make Decisions At Scale Through AI With Numerous AI’s Spreadsheet AI Tool
What is Data Cleaning? Why It’s Essential for Accurate Analysis
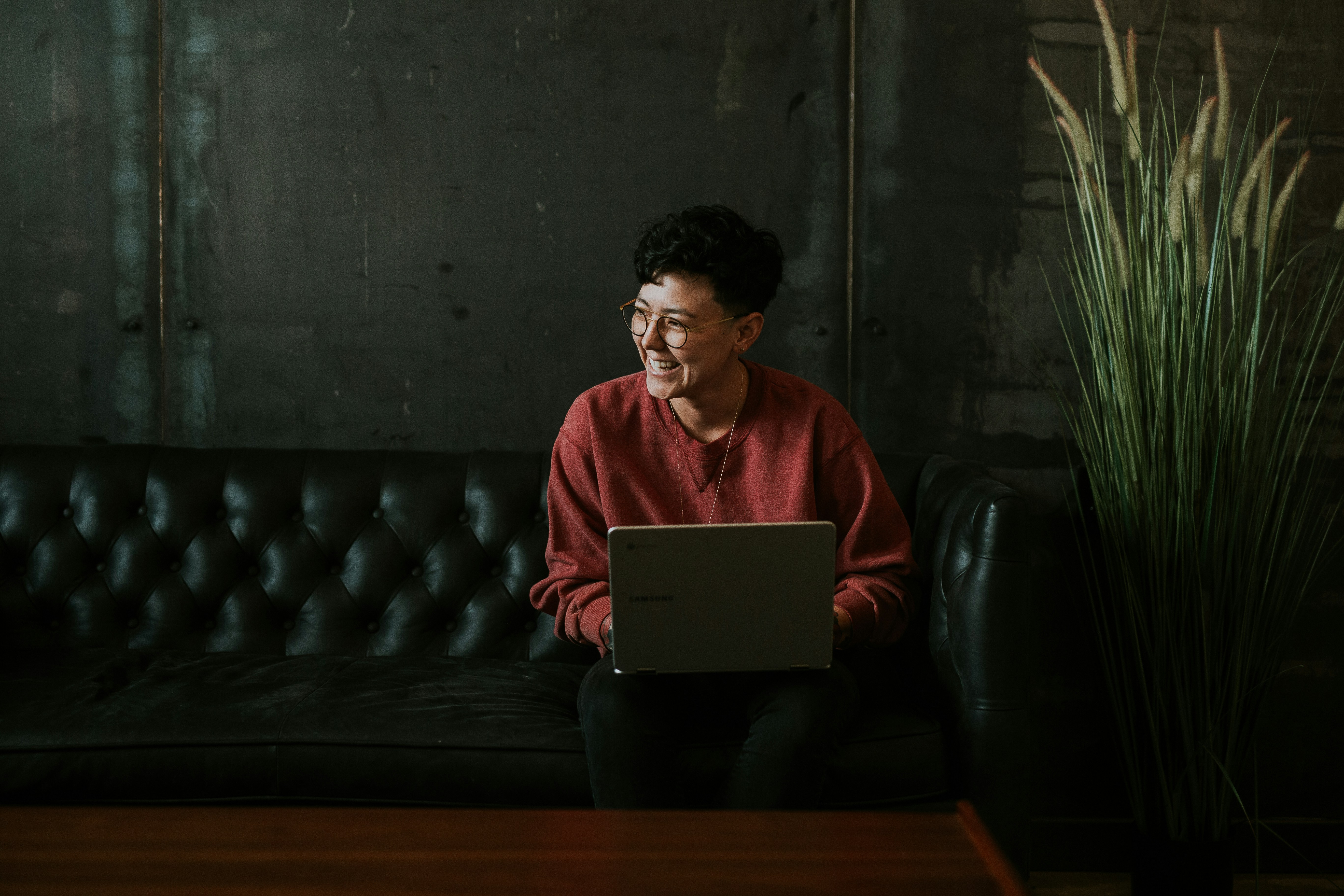
Data cleaning (also called data scrubbing or data cleansing) is identifying, correcting, and removing errors or inconsistencies in a dataset to ensure it is accurate, complete, and ready for analysis. It is a critical step in data preparation that helps businesses, researchers, and analysts make reliable decisions based on clean, structured data.
At its core, data cleaning involves:
Fixing missing or incorrect values
Standardizing formatting across datasets
Removing duplicate records
Eliminating spelling, naming conventions, or categorization inconsistencies
Validating data against rules and benchmarks.
Organizations risk basing their decisions on inaccurate or misleading information without proper data cleaning, leading to financial losses, incorrect insights, and operational inefficiencies.
Why is Data Cleaning Important?
Data is one of the most valuable assets for any business. However, raw data is often messy due to errors from human entry, system migrations, or external data imports. If left uncleaned, this dirty data can cause:
A. Poor Decision-Making
Unclean data leads to inaccurate reports, incorrect trends, and flawed insights.
Example: A retail company analyzing customer demographics might draw erroneous conclusions if duplicate records cause one customer to be counted multiple times.
B. Financial & Reputational Damage
Dirty data can lead to mispriced products, wrong customer segmentation, or incorrect invoices being sent.
Example: An eCommerce company might lose revenue by offering discounts to the wrong customer segment due to poor data categorization.
C. Decreased Productivity & Increased Manual Work
Without automated data cleaning, employees spend hours manually fixing errors.
Example: A data analyst may waste 40%–60% of their time correcting messy datasets instead of focusing on insights.
D. Compliance & Regulatory Risks
Many industries (e.g., healthcare, finance, legal) must maintain clean data for regulatory compliance.
Example: A financial institution might face penalties if incorrect customer data affects KYC (Know Your Customer) compliance.
By implementing AI-driven data cleaning tools, businesses can ensure that data is always accurate, consistent, and structured—enabling better decision-making, operational efficiency, and compliance.
Common Data Issues That Require Cleaning
Raw data is rarely perfect. It often contains errors, inconsistencies, and formatting issues that must be corrected before analysis. The most common issues include:
A. Missing Data (Incomplete Records) Problem
Gaps in datasets where essential values (e.g., customer emails, product prices, timestamps) are missing.
Example: A sales report's "Total Revenue" column is missing values, making it impossible to calculate accurate profits.
Solution: AI-powered tools can fill in missing values using machine learning predictions or statistical methods (e.g., median imputation).
B. Duplicate Data (Repeated Entries) Problem
The same customer, transaction, or product appears multiple times in a dataset, leading to overestimated counts.
Example: A customer appears in the CRM three times with slightly different name spellings (John Doe, Jon Doe, J. Doe), leading to inaccurate customer reports.
Solution: AI-driven de-duplication algorithms identify and merge duplicate records automatically.
C. Inconsistent Formatting Problem
Data is stored in different formats, making it hard to standardize and analyze.
Example: Dates appear in multiple formats (MM/DD/YYYY, DD/MM/YYYY, YYYY-MM-DD) across different regions, confusing time-sensitive analysis.
Solution: AI-powered data cleaning tools can automatically standardize date formats across the dataset.
D. Incorrect Data Entries (Typos, Spelling Errors, Mismatched Fields)
Problem: Errors caused by manual data entry mistakes or poorly formatted imports.
Example: A product catalog has inconsistent naming conventions: "Nike Air Max 2025," "nike_airmax_2025," and "Nike Air Max 25." This makes it impossible to track sales of the same product accurately.
Solution: AI-driven text standardization tools fix inconsistencies and align product names automatically.
E. Outdated or Irrelevant Data Problem
Datasets contain old or obsolete information.
Example: A customer database still contains records of users who haven't purchased in over 10 years, distorting marketing campaign results.
Solution: AI-powered tools can detect outdated records and suggest actions such as archiving or removal.
How AI is Transforming Data Cleaning in 2025
Traditionally, data cleaning was a manual, time-consuming process requiring hours of work from data analysts. However, AI-powered automation tools like Numerous have changed the game by making data cleaning faster, more innovative, and more efficient.
A. AI-Powered Automation Eliminates Repetitive Tasks
Instead of manually fixing errors, AI can automatically detect and correct missing values, identify duplicate entries, and intelligently merge them. It can also standardize formatting (dates, numbers, capitalization, etc.).
B. AI Predicts & Fills in Missing Data
Using machine learning, AI can Predict missing values based on existing patterns. Auto-fill incomplete records to maintain data integrity. Suggest corrections based on previous clean data.
C. AI Can Clean Data in Real-Time
With tools like Numerous, AI can continuously monitor and clean incoming data, ensuring that mistakes are fixed instantly instead of accumulating over time.
D. AI Integration in Spreadsheets Saves Time & Effort
AI-powered spreadsheet tools, like Numerous, enable users to clean data directly in Excel or Google Sheets using a simple prompt.
Instead of spending hours on formulas, users can type:
"Find and delete duplicate rows,"
"Standardize phone numbers to international format,"
"Identify and correct inconsistent product names."
Numerous then execute these tasks instantly, saving hours of manual work.
Related Reading
• Data Cleaning Process
• Data Cleaning Example
• How to Validate Data
• Data Validation Techniques
• Data Cleaning Best Practices
• Data Validation Best Practices
• Data Cleaning Example
How to Write AI Prompts for Data Cleaning (Step-by-Step Guide)

AI prompts are natural language commands or questions that inform an AI tool of precisely what to do. Users can describe their intent in plain language rather than relying on formulas, scripts, or manual inputs, and the AI automates the task.
For example, without AI, cleaning up a date format in a spreadsheet requires using a complex formula like =TEXT(A2, "YYYY-MM-DD"). This formula manually converts a date to a standardized format. With AI, you simply type: "Convert all dates in Column A to YYYY-MM-DD format." The AI automatically detects and applies the formatting to the entire column.
Best Practices for Writing Effective AI Prompts
To ensure AI understands and executes your request correctly, follow these best practices:
Be Clear and Specific
AI tools work best with precise instructions. Instead of saying, "Clean up my data," specify precisely what needs cleaning.
Good Example: "Find and remove duplicate email addresses in Column C while keeping the first occurrence."
Bad Example: "Remove duplicates." (Too vague—what column? Which duplicate should be kept?)
Use Action Words
AI responds well to clear, direct actions like convert, clean, find, remove, standardize, categorize, or format.
Good Example: "Find and replace all instances of 'N/A' in Column B with 'Not Available'."
Bad Example: "Change data." (It is too vague. What data needs changing? How should it be changed?)
Specify Columns or Data Ranges
AI works on entire datasets, so specifying columns or ranges prevents mistakes.
Good Example: "Standardize capitalization in Column D so that each word starts with a capital letter."
Bad Example: "Fix capitalization." (Fix where? Capitalization in what way?)
Provide Formatting Details
If formatting is required, specify the desired format.
Good Example: "Convert all dates in Column A to YYYY-MM-DD format."
Bad Example: "Fix the date format." (What format should it be?)
Define Conditions for Cleaning Tasks
If conditional logic applies, include it in the prompt.
Good Example: "Remove duplicate rows only if the values in Column B and Column C are identical."
Bad Example: "Remove some duplicates." (How should AI determine which to remove?)
Use Natural Language, But Avoid Unnecessary Words
AI can process natural language, but overly conversational prompts may reduce accuracy.
Good Example: "Identify and highlight all cells in Column E that contain numbers lower than 10."
Bad Example: "Hey AI, can you please take a look at Column E and tell me which ones have numbers under 10? Thanks!" (Too wordy—keep it structured.)
How to Structure AI Prompts for Data Cleaning
Basic Structure of an AI Prompt
To write a clear, effective prompt, follow this simple structure: Action + Data Range + Condition (if needed) + Formatting (if required)
Examples of Well-Structured AI Prompts
Handling Missing Data: "Fill all missing values in Column D using the column's median value." "Identify and highlight all empty cells in this dataset."
Detecting & Removing Duplicates: "Find and remove duplicate rows in Column C while keeping the first occurrence." "Merge duplicate customer names in Column A, keeping the most recent record."
Standardizing Formatting: "Ensure all email addresses in Column F are in lowercase." "Convert all text in Column B to title case (capitalize first letter of each word)."
Text Cleaning & Categorization: "Replace all instances of 'N/A' with 'Not Available' in Column G." "Classify customer reviews in Column H as 'Positive,' 'Neutral,' or 'Negative' based on sentiment analysis."
Outlier & Error Detection: "Flag all sales amounts in Column E that are above $10,000 as outliers." "Identify and correct any negative numbers in the 'Revenue' column."
Using AI Prompts in Spreadsheets (Google Sheets & Excel)
Step-by-Step Guide to Implement AI Prompts in Numerous (Google Sheets & Excel)
Open Google Sheets or Microsoft Excel. Enable Numerous AI (if installed as an add-on or extension). Type your AI prompt into the input box or command section. Example: "Find and delete duplicate product codes in Column B while keeping the first occurrence." Review the AI’s suggestions. Click 'Apply' to execute the data cleaning process automatically. This removes the need for formulas or scripts, allowing users to instantly clean, standardize, and organize data.
Related Reading
• Challenges of Data Cleaning
• Automated Data Validation
• AI Data Validation
• Customer Data Cleansing
• Benefits of Using AI for Data Cleaning
• Data Cleansing Strategy
• Data Cleaning Checklist
• AI Data Cleaning Tool
• Machine Learning Data Cleaning
• Challenges of AI Data Cleaning
• Data Cleaning Methods
25 Best AI Prompts for Data Cleaning
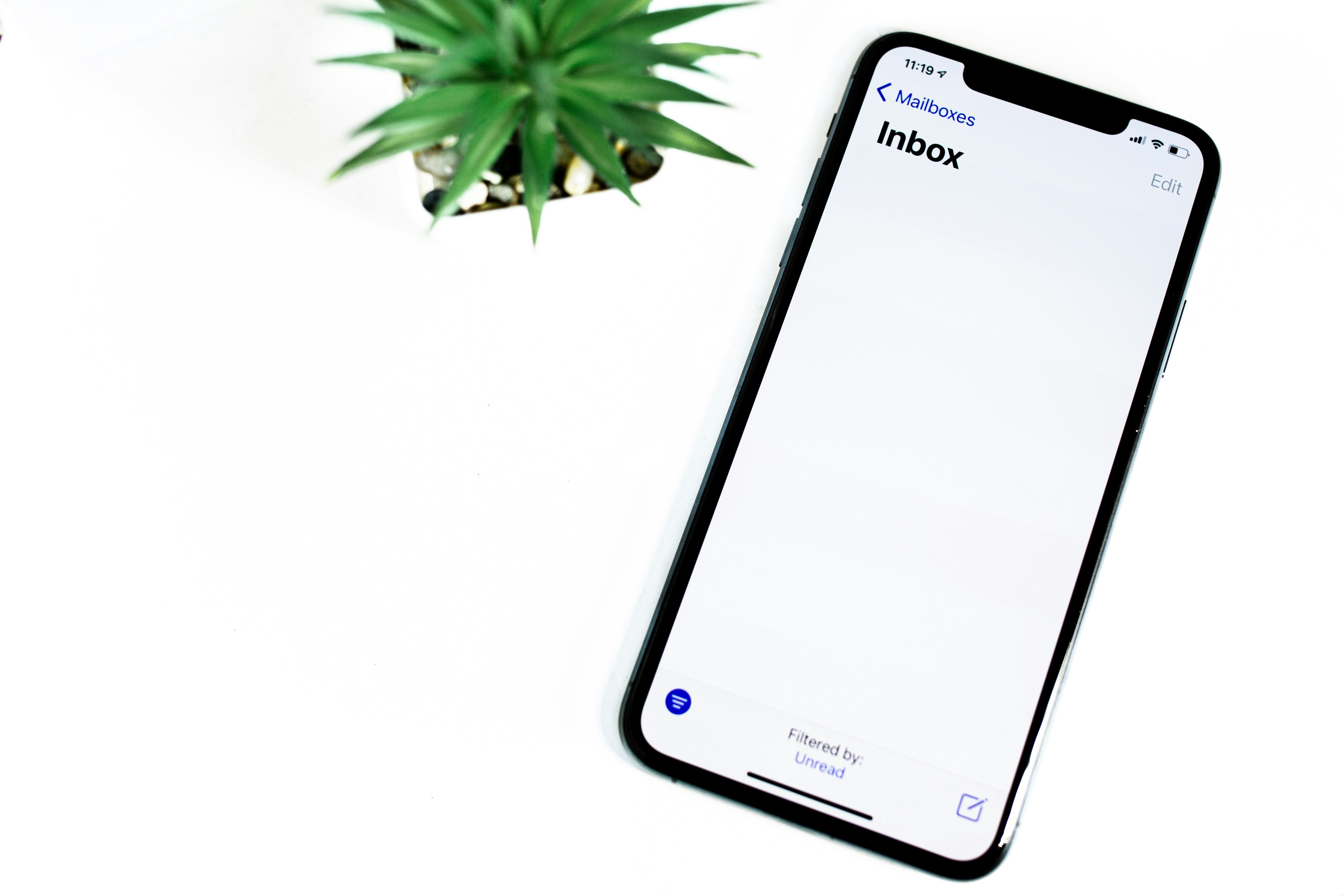
A. Handling Missing Data
Missing data can distort analysis and make datasets unreliable. The best AI prompts for handling missing data automatically identify, fill, or manage missing values.
"Identify and highlight all missing values in this dataset." This prompt uncovers all the gaps and helps you visualize where the problems are.
"Fill missing numerical values using the median of the column." This prompt fills gaps with the median value, often the best choice for avoiding bias in datasets with outliers.
"Fill missing numerical values using linear interpolation." This prompt fills gaps with values based on existing trends, ensuring a smooth transition between data points.
"Replace all missing text values with ‘Not Available’." This prompt standardizes missing text values to avoid confusion during analysis.
"Suggest the most likely values for missing entries based on existing patterns." This prompt uncovers existing patterns and helps you fill gaps with the most relevant values.
B. Detecting & Removing Duplicate Entries
Duplicate records inflate counts, cause errors in analysis, and mislead decision-making. The best AI prompts for cleaning duplicates efficiently detect and remove redundant records from datasets.
"Find and delete duplicate rows while keeping the first occurrence." This prompt uncovers exact duplicate entries and removes them automatically to streamline your dataset.
"Identify near-duplicate customer records and suggest merging strategies." This prompt uncovers variations of the same record that can mislead analysis and identifies ways to merge them.
"Highlight rows where the same email appears more than once." This prompt finds multiple occurrences of the same email address to help you investigate and remove redundant entries.
"Remove duplicate entries from this dataset while preserving the most recent record." This prompt identifies and deletes duplicate rows while ensuring you keep the latest version of the record.
"Find duplicate product names that have slight spelling variations." This prompt locates product names that are likely the same but have minor spelling differences, making them appear unique.
C. Standardizing Formatting Across Datasets
Inconsistent formatting makes data look unstructured and difficult to analyze. The best AI prompts for standardizing formatting across datasets ensure the data looks uniform.
"Convert all dates to the YYYY-MM-DD format." This prompt eliminates variations in date formatting by converting them all to the ISO standard, which is universally recognized.
“Ensure phone numbers follow international formatting (+CountryCode Number)." This prompt identifies and corrects any inconsistencies in phone number formatting so that all entries follow a standard format.
"Standardize all product names to Title Case formatting." This prompt corrects any inconsistencies in capitalizing product names and ensures they all follow Title Case formatting.
"Ensure all text entries in Column B follow sentence case." This prompt identifies text entries that are not in the proper case and corrects them automatically.
"Remove all leading and trailing spaces from text entries." This prompt eliminates any extra spaces in text entries that can cause errors during analysis.
D. Text Cleaning & Data Categorization
Unstructured text can cause inconsistencies in reporting and analytics. The best AI prompts for cleaning text data can structure, categorize, and organize text-based data.
"Standardize all company names by removing extra spaces and capitalization inconsistencies." This prompt cleans company names to ensure consistency for reporting and analysis.
"Identify and correct common spelling errors in this dataset." This prompt finds and fixes misspelled words in your dataset to improve overall quality.
“Classify customer reviews as Positive, Neutral, or Negative based on sentiment analysis." This prompt uses AI to understand the context of text entries and automatically categorize them into predefined groups.
"Extract only the domain names from a list of email addresses." This prompt removes all unnecessary text from your dataset and organizes the data into a more usable format.
"Identify and categorize product descriptions into predefined categories." This prompt analyzes product descriptions and uses existing rules to classify them for better organization.
E. Detecting Outliers & Errors in Data
Outliers can skew data insights and create misleading trends. The best AI prompts for detecting outliers automatically identify and correct such anomalies.
"Find all numerical values significantly higher or lower than the average." This prompt uncovers rogue data points that fall far outside the normal range and may need to be corrected or removed.
"Detect incorrect numerical values that do not match expected patterns (e.g., negative prices in a sales dataset)." This prompt identifies data points that are likely erroneous and need to be investigated before analysis.
"Flag inconsistent pricing for identical products." This prompt finds pricing variations for the same product that may result from data entry errors.
"Identify incorrectly formatted email addresses." This prompt locates email entries that do not follow standard formatting so you can fix them before analysis.
"Detect abnormal transaction amounts based on historical trends." This prompt analyzes past transaction data to establish a range of expected values and uncovers any recent entries that fall outside this norm.
Make Decisions At Scale Through AI With Numerous AI’s Spreadsheet AI Tool
AI prompts for data cleaning are designed to help users tackle the tedious, repetitive tasks accompanying large-scale data cleaning. This can include identifying and correcting errors within datasets and removing duplicate entries. Using these brilliant prompts, users can automate many of their data-cleaning tasks to help reduce human error and make the process far more efficient.
Numerous is an AI-Powered tool that enables content marketers, Ecommerce businesses, and more to do tasks many times over through AI, like writing SEO blog posts, generating hashtags, mass categorizing products with sentiment analysis and classification, and many more things by simply dragging down a cell in a spreadsheet. With a simple prompt, Numerous returns any spreadsheet function, simple or complex, within seconds.
The capabilities of Numerous are endless. It is versatile and can be used with Microsoft Excel and Google Sheets. Get started today with Numerous.ai so that you can make business decisions at scale using AI in both Google Sheets and Microsoft Excel. Learn more about how you can 10x your marketing efforts with Numerous’s ChatGPT for Spreadsheets tool.
Related Reading
• Talend Alternatives
• Informatica Alternatives
• Data Cleansing Tools
• AI vs Traditional Data Cleaning Methods
• Alteryx Alternative
• Data Validation Tools
Cleaning data is like cleaning out your garage. At first, it seems like a simple task. But once you start, you realize it's much more complex than you thought. Before long, you uncover forgotten junk and hidden treasures that must be tossed, repaired, or organized. In the case of data, these items are errors and anomalies, some of which can be fixed with a simple delete or find and replace command, while others demand more intricate attention.
And just like there’s no telling how long a garage cleaning might take, the same goes for data cleaning techniques. You can't predict how long it will take until you get into the task and figure out what’s happening. The good news is that you can speed up the process and make it less painful with the right tools. This blog will introduce you to 25 of the best secret AI prompts for data cleaning you need to know today to help you uncover and organize your data’s hidden treasures.
Numerous spreadsheet AI tool is among the best for learning and implementing these prompts. It uses open AI technology to help you quickly create data-cleaning tasks and automate the process to get to your clean data faster.
Table of Content
What is Data Cleaning? Why It’s Essential for Accurate Analysis
How to Write AI Prompts for Data Cleaning (Step-by-Step Guide)
Make Decisions At Scale Through AI With Numerous AI’s Spreadsheet AI Tool
What is Data Cleaning? Why It’s Essential for Accurate Analysis
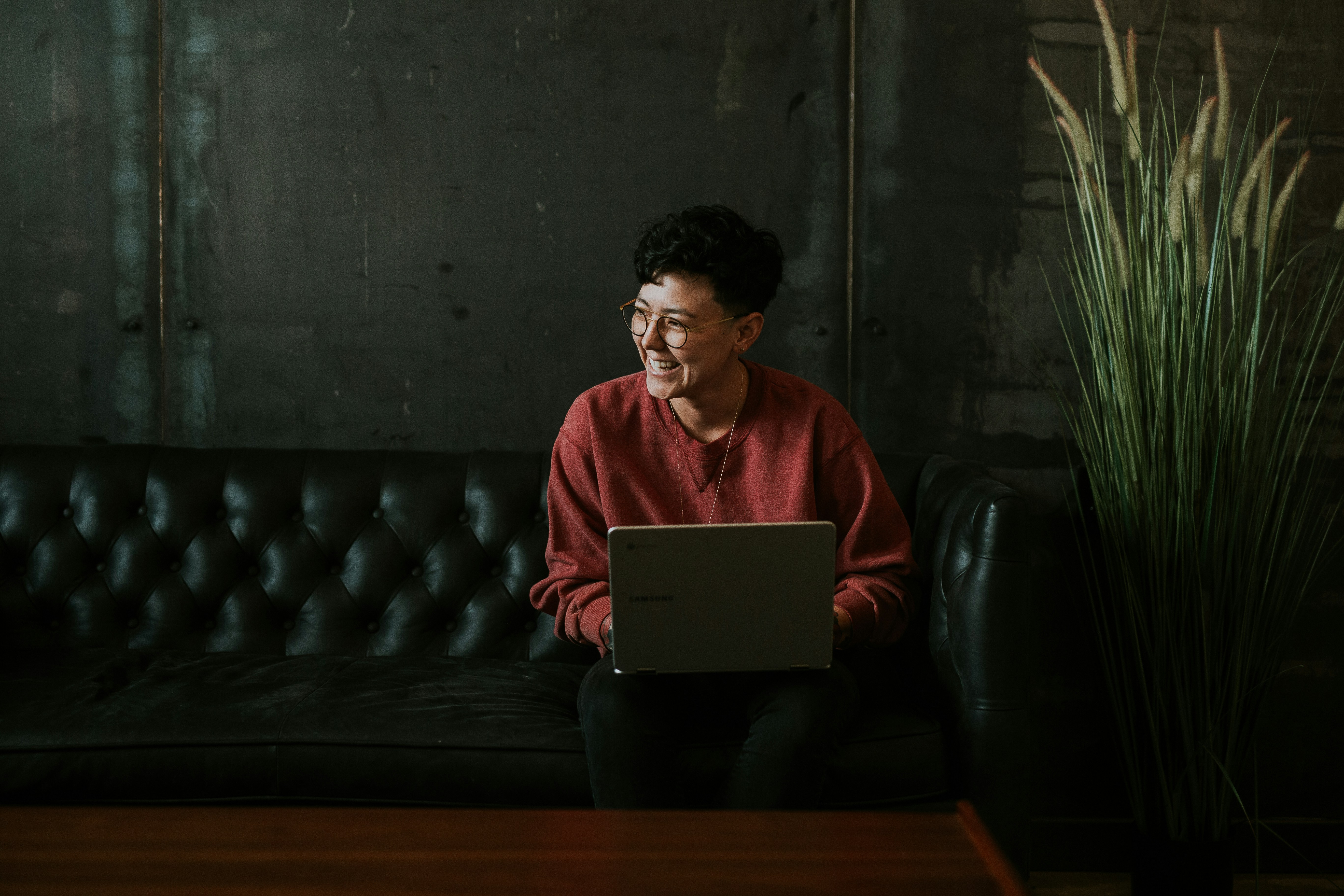
Data cleaning (also called data scrubbing or data cleansing) is identifying, correcting, and removing errors or inconsistencies in a dataset to ensure it is accurate, complete, and ready for analysis. It is a critical step in data preparation that helps businesses, researchers, and analysts make reliable decisions based on clean, structured data.
At its core, data cleaning involves:
Fixing missing or incorrect values
Standardizing formatting across datasets
Removing duplicate records
Eliminating spelling, naming conventions, or categorization inconsistencies
Validating data against rules and benchmarks.
Organizations risk basing their decisions on inaccurate or misleading information without proper data cleaning, leading to financial losses, incorrect insights, and operational inefficiencies.
Why is Data Cleaning Important?
Data is one of the most valuable assets for any business. However, raw data is often messy due to errors from human entry, system migrations, or external data imports. If left uncleaned, this dirty data can cause:
A. Poor Decision-Making
Unclean data leads to inaccurate reports, incorrect trends, and flawed insights.
Example: A retail company analyzing customer demographics might draw erroneous conclusions if duplicate records cause one customer to be counted multiple times.
B. Financial & Reputational Damage
Dirty data can lead to mispriced products, wrong customer segmentation, or incorrect invoices being sent.
Example: An eCommerce company might lose revenue by offering discounts to the wrong customer segment due to poor data categorization.
C. Decreased Productivity & Increased Manual Work
Without automated data cleaning, employees spend hours manually fixing errors.
Example: A data analyst may waste 40%–60% of their time correcting messy datasets instead of focusing on insights.
D. Compliance & Regulatory Risks
Many industries (e.g., healthcare, finance, legal) must maintain clean data for regulatory compliance.
Example: A financial institution might face penalties if incorrect customer data affects KYC (Know Your Customer) compliance.
By implementing AI-driven data cleaning tools, businesses can ensure that data is always accurate, consistent, and structured—enabling better decision-making, operational efficiency, and compliance.
Common Data Issues That Require Cleaning
Raw data is rarely perfect. It often contains errors, inconsistencies, and formatting issues that must be corrected before analysis. The most common issues include:
A. Missing Data (Incomplete Records) Problem
Gaps in datasets where essential values (e.g., customer emails, product prices, timestamps) are missing.
Example: A sales report's "Total Revenue" column is missing values, making it impossible to calculate accurate profits.
Solution: AI-powered tools can fill in missing values using machine learning predictions or statistical methods (e.g., median imputation).
B. Duplicate Data (Repeated Entries) Problem
The same customer, transaction, or product appears multiple times in a dataset, leading to overestimated counts.
Example: A customer appears in the CRM three times with slightly different name spellings (John Doe, Jon Doe, J. Doe), leading to inaccurate customer reports.
Solution: AI-driven de-duplication algorithms identify and merge duplicate records automatically.
C. Inconsistent Formatting Problem
Data is stored in different formats, making it hard to standardize and analyze.
Example: Dates appear in multiple formats (MM/DD/YYYY, DD/MM/YYYY, YYYY-MM-DD) across different regions, confusing time-sensitive analysis.
Solution: AI-powered data cleaning tools can automatically standardize date formats across the dataset.
D. Incorrect Data Entries (Typos, Spelling Errors, Mismatched Fields)
Problem: Errors caused by manual data entry mistakes or poorly formatted imports.
Example: A product catalog has inconsistent naming conventions: "Nike Air Max 2025," "nike_airmax_2025," and "Nike Air Max 25." This makes it impossible to track sales of the same product accurately.
Solution: AI-driven text standardization tools fix inconsistencies and align product names automatically.
E. Outdated or Irrelevant Data Problem
Datasets contain old or obsolete information.
Example: A customer database still contains records of users who haven't purchased in over 10 years, distorting marketing campaign results.
Solution: AI-powered tools can detect outdated records and suggest actions such as archiving or removal.
How AI is Transforming Data Cleaning in 2025
Traditionally, data cleaning was a manual, time-consuming process requiring hours of work from data analysts. However, AI-powered automation tools like Numerous have changed the game by making data cleaning faster, more innovative, and more efficient.
A. AI-Powered Automation Eliminates Repetitive Tasks
Instead of manually fixing errors, AI can automatically detect and correct missing values, identify duplicate entries, and intelligently merge them. It can also standardize formatting (dates, numbers, capitalization, etc.).
B. AI Predicts & Fills in Missing Data
Using machine learning, AI can Predict missing values based on existing patterns. Auto-fill incomplete records to maintain data integrity. Suggest corrections based on previous clean data.
C. AI Can Clean Data in Real-Time
With tools like Numerous, AI can continuously monitor and clean incoming data, ensuring that mistakes are fixed instantly instead of accumulating over time.
D. AI Integration in Spreadsheets Saves Time & Effort
AI-powered spreadsheet tools, like Numerous, enable users to clean data directly in Excel or Google Sheets using a simple prompt.
Instead of spending hours on formulas, users can type:
"Find and delete duplicate rows,"
"Standardize phone numbers to international format,"
"Identify and correct inconsistent product names."
Numerous then execute these tasks instantly, saving hours of manual work.
Related Reading
• Data Cleaning Process
• Data Cleaning Example
• How to Validate Data
• Data Validation Techniques
• Data Cleaning Best Practices
• Data Validation Best Practices
• Data Cleaning Example
How to Write AI Prompts for Data Cleaning (Step-by-Step Guide)

AI prompts are natural language commands or questions that inform an AI tool of precisely what to do. Users can describe their intent in plain language rather than relying on formulas, scripts, or manual inputs, and the AI automates the task.
For example, without AI, cleaning up a date format in a spreadsheet requires using a complex formula like =TEXT(A2, "YYYY-MM-DD"). This formula manually converts a date to a standardized format. With AI, you simply type: "Convert all dates in Column A to YYYY-MM-DD format." The AI automatically detects and applies the formatting to the entire column.
Best Practices for Writing Effective AI Prompts
To ensure AI understands and executes your request correctly, follow these best practices:
Be Clear and Specific
AI tools work best with precise instructions. Instead of saying, "Clean up my data," specify precisely what needs cleaning.
Good Example: "Find and remove duplicate email addresses in Column C while keeping the first occurrence."
Bad Example: "Remove duplicates." (Too vague—what column? Which duplicate should be kept?)
Use Action Words
AI responds well to clear, direct actions like convert, clean, find, remove, standardize, categorize, or format.
Good Example: "Find and replace all instances of 'N/A' in Column B with 'Not Available'."
Bad Example: "Change data." (It is too vague. What data needs changing? How should it be changed?)
Specify Columns or Data Ranges
AI works on entire datasets, so specifying columns or ranges prevents mistakes.
Good Example: "Standardize capitalization in Column D so that each word starts with a capital letter."
Bad Example: "Fix capitalization." (Fix where? Capitalization in what way?)
Provide Formatting Details
If formatting is required, specify the desired format.
Good Example: "Convert all dates in Column A to YYYY-MM-DD format."
Bad Example: "Fix the date format." (What format should it be?)
Define Conditions for Cleaning Tasks
If conditional logic applies, include it in the prompt.
Good Example: "Remove duplicate rows only if the values in Column B and Column C are identical."
Bad Example: "Remove some duplicates." (How should AI determine which to remove?)
Use Natural Language, But Avoid Unnecessary Words
AI can process natural language, but overly conversational prompts may reduce accuracy.
Good Example: "Identify and highlight all cells in Column E that contain numbers lower than 10."
Bad Example: "Hey AI, can you please take a look at Column E and tell me which ones have numbers under 10? Thanks!" (Too wordy—keep it structured.)
How to Structure AI Prompts for Data Cleaning
Basic Structure of an AI Prompt
To write a clear, effective prompt, follow this simple structure: Action + Data Range + Condition (if needed) + Formatting (if required)
Examples of Well-Structured AI Prompts
Handling Missing Data: "Fill all missing values in Column D using the column's median value." "Identify and highlight all empty cells in this dataset."
Detecting & Removing Duplicates: "Find and remove duplicate rows in Column C while keeping the first occurrence." "Merge duplicate customer names in Column A, keeping the most recent record."
Standardizing Formatting: "Ensure all email addresses in Column F are in lowercase." "Convert all text in Column B to title case (capitalize first letter of each word)."
Text Cleaning & Categorization: "Replace all instances of 'N/A' with 'Not Available' in Column G." "Classify customer reviews in Column H as 'Positive,' 'Neutral,' or 'Negative' based on sentiment analysis."
Outlier & Error Detection: "Flag all sales amounts in Column E that are above $10,000 as outliers." "Identify and correct any negative numbers in the 'Revenue' column."
Using AI Prompts in Spreadsheets (Google Sheets & Excel)
Step-by-Step Guide to Implement AI Prompts in Numerous (Google Sheets & Excel)
Open Google Sheets or Microsoft Excel. Enable Numerous AI (if installed as an add-on or extension). Type your AI prompt into the input box or command section. Example: "Find and delete duplicate product codes in Column B while keeping the first occurrence." Review the AI’s suggestions. Click 'Apply' to execute the data cleaning process automatically. This removes the need for formulas or scripts, allowing users to instantly clean, standardize, and organize data.
Related Reading
• Challenges of Data Cleaning
• Automated Data Validation
• AI Data Validation
• Customer Data Cleansing
• Benefits of Using AI for Data Cleaning
• Data Cleansing Strategy
• Data Cleaning Checklist
• AI Data Cleaning Tool
• Machine Learning Data Cleaning
• Challenges of AI Data Cleaning
• Data Cleaning Methods
25 Best AI Prompts for Data Cleaning
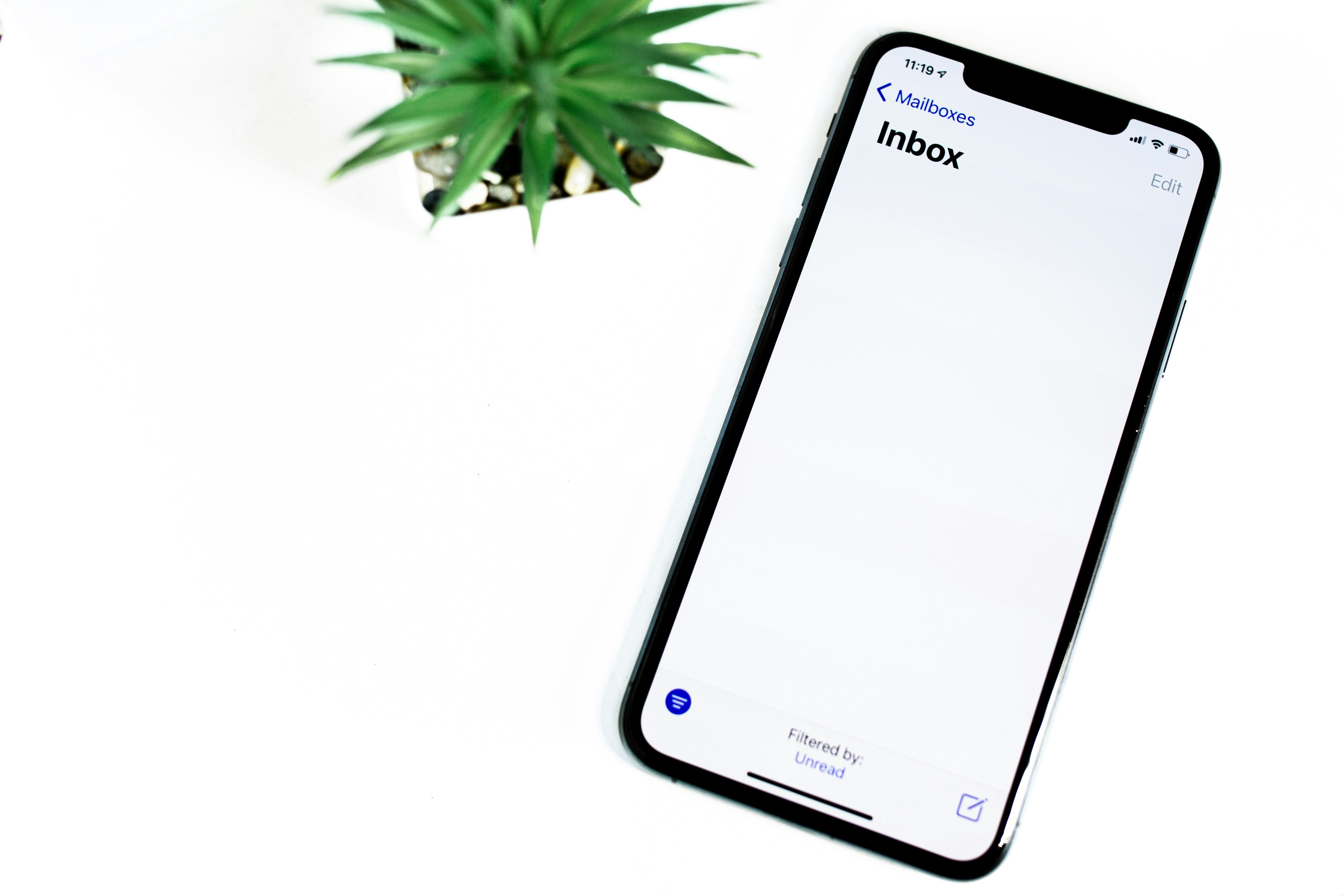
A. Handling Missing Data
Missing data can distort analysis and make datasets unreliable. The best AI prompts for handling missing data automatically identify, fill, or manage missing values.
"Identify and highlight all missing values in this dataset." This prompt uncovers all the gaps and helps you visualize where the problems are.
"Fill missing numerical values using the median of the column." This prompt fills gaps with the median value, often the best choice for avoiding bias in datasets with outliers.
"Fill missing numerical values using linear interpolation." This prompt fills gaps with values based on existing trends, ensuring a smooth transition between data points.
"Replace all missing text values with ‘Not Available’." This prompt standardizes missing text values to avoid confusion during analysis.
"Suggest the most likely values for missing entries based on existing patterns." This prompt uncovers existing patterns and helps you fill gaps with the most relevant values.
B. Detecting & Removing Duplicate Entries
Duplicate records inflate counts, cause errors in analysis, and mislead decision-making. The best AI prompts for cleaning duplicates efficiently detect and remove redundant records from datasets.
"Find and delete duplicate rows while keeping the first occurrence." This prompt uncovers exact duplicate entries and removes them automatically to streamline your dataset.
"Identify near-duplicate customer records and suggest merging strategies." This prompt uncovers variations of the same record that can mislead analysis and identifies ways to merge them.
"Highlight rows where the same email appears more than once." This prompt finds multiple occurrences of the same email address to help you investigate and remove redundant entries.
"Remove duplicate entries from this dataset while preserving the most recent record." This prompt identifies and deletes duplicate rows while ensuring you keep the latest version of the record.
"Find duplicate product names that have slight spelling variations." This prompt locates product names that are likely the same but have minor spelling differences, making them appear unique.
C. Standardizing Formatting Across Datasets
Inconsistent formatting makes data look unstructured and difficult to analyze. The best AI prompts for standardizing formatting across datasets ensure the data looks uniform.
"Convert all dates to the YYYY-MM-DD format." This prompt eliminates variations in date formatting by converting them all to the ISO standard, which is universally recognized.
“Ensure phone numbers follow international formatting (+CountryCode Number)." This prompt identifies and corrects any inconsistencies in phone number formatting so that all entries follow a standard format.
"Standardize all product names to Title Case formatting." This prompt corrects any inconsistencies in capitalizing product names and ensures they all follow Title Case formatting.
"Ensure all text entries in Column B follow sentence case." This prompt identifies text entries that are not in the proper case and corrects them automatically.
"Remove all leading and trailing spaces from text entries." This prompt eliminates any extra spaces in text entries that can cause errors during analysis.
D. Text Cleaning & Data Categorization
Unstructured text can cause inconsistencies in reporting and analytics. The best AI prompts for cleaning text data can structure, categorize, and organize text-based data.
"Standardize all company names by removing extra spaces and capitalization inconsistencies." This prompt cleans company names to ensure consistency for reporting and analysis.
"Identify and correct common spelling errors in this dataset." This prompt finds and fixes misspelled words in your dataset to improve overall quality.
“Classify customer reviews as Positive, Neutral, or Negative based on sentiment analysis." This prompt uses AI to understand the context of text entries and automatically categorize them into predefined groups.
"Extract only the domain names from a list of email addresses." This prompt removes all unnecessary text from your dataset and organizes the data into a more usable format.
"Identify and categorize product descriptions into predefined categories." This prompt analyzes product descriptions and uses existing rules to classify them for better organization.
E. Detecting Outliers & Errors in Data
Outliers can skew data insights and create misleading trends. The best AI prompts for detecting outliers automatically identify and correct such anomalies.
"Find all numerical values significantly higher or lower than the average." This prompt uncovers rogue data points that fall far outside the normal range and may need to be corrected or removed.
"Detect incorrect numerical values that do not match expected patterns (e.g., negative prices in a sales dataset)." This prompt identifies data points that are likely erroneous and need to be investigated before analysis.
"Flag inconsistent pricing for identical products." This prompt finds pricing variations for the same product that may result from data entry errors.
"Identify incorrectly formatted email addresses." This prompt locates email entries that do not follow standard formatting so you can fix them before analysis.
"Detect abnormal transaction amounts based on historical trends." This prompt analyzes past transaction data to establish a range of expected values and uncovers any recent entries that fall outside this norm.
Make Decisions At Scale Through AI With Numerous AI’s Spreadsheet AI Tool
AI prompts for data cleaning are designed to help users tackle the tedious, repetitive tasks accompanying large-scale data cleaning. This can include identifying and correcting errors within datasets and removing duplicate entries. Using these brilliant prompts, users can automate many of their data-cleaning tasks to help reduce human error and make the process far more efficient.
Numerous is an AI-Powered tool that enables content marketers, Ecommerce businesses, and more to do tasks many times over through AI, like writing SEO blog posts, generating hashtags, mass categorizing products with sentiment analysis and classification, and many more things by simply dragging down a cell in a spreadsheet. With a simple prompt, Numerous returns any spreadsheet function, simple or complex, within seconds.
The capabilities of Numerous are endless. It is versatile and can be used with Microsoft Excel and Google Sheets. Get started today with Numerous.ai so that you can make business decisions at scale using AI in both Google Sheets and Microsoft Excel. Learn more about how you can 10x your marketing efforts with Numerous’s ChatGPT for Spreadsheets tool.
Related Reading
• Talend Alternatives
• Informatica Alternatives
• Data Cleansing Tools
• AI vs Traditional Data Cleaning Methods
• Alteryx Alternative
• Data Validation Tools
Cleaning data is like cleaning out your garage. At first, it seems like a simple task. But once you start, you realize it's much more complex than you thought. Before long, you uncover forgotten junk and hidden treasures that must be tossed, repaired, or organized. In the case of data, these items are errors and anomalies, some of which can be fixed with a simple delete or find and replace command, while others demand more intricate attention.
And just like there’s no telling how long a garage cleaning might take, the same goes for data cleaning techniques. You can't predict how long it will take until you get into the task and figure out what’s happening. The good news is that you can speed up the process and make it less painful with the right tools. This blog will introduce you to 25 of the best secret AI prompts for data cleaning you need to know today to help you uncover and organize your data’s hidden treasures.
Numerous spreadsheet AI tool is among the best for learning and implementing these prompts. It uses open AI technology to help you quickly create data-cleaning tasks and automate the process to get to your clean data faster.
Table of Content
What is Data Cleaning? Why It’s Essential for Accurate Analysis
How to Write AI Prompts for Data Cleaning (Step-by-Step Guide)
Make Decisions At Scale Through AI With Numerous AI’s Spreadsheet AI Tool
What is Data Cleaning? Why It’s Essential for Accurate Analysis
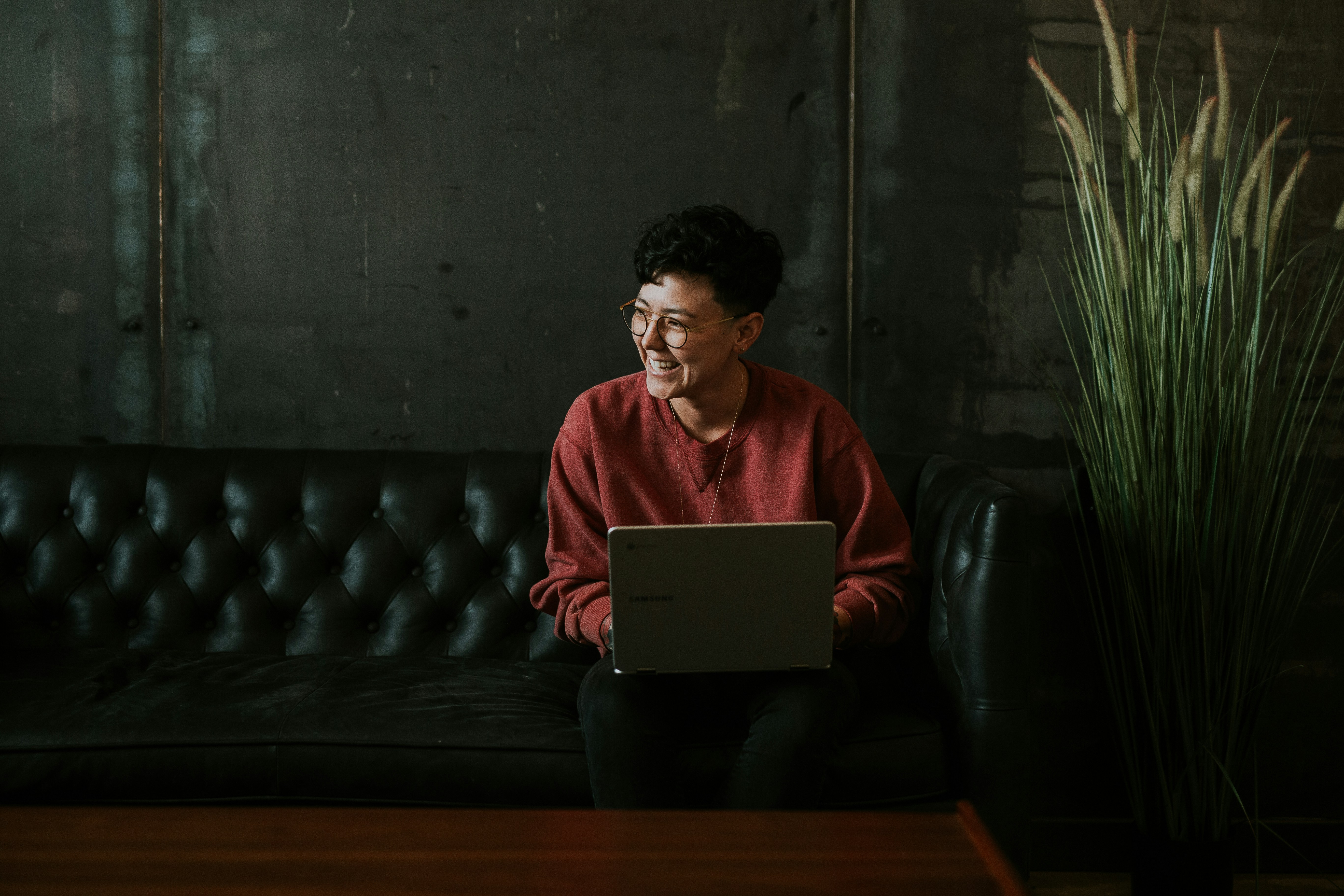
Data cleaning (also called data scrubbing or data cleansing) is identifying, correcting, and removing errors or inconsistencies in a dataset to ensure it is accurate, complete, and ready for analysis. It is a critical step in data preparation that helps businesses, researchers, and analysts make reliable decisions based on clean, structured data.
At its core, data cleaning involves:
Fixing missing or incorrect values
Standardizing formatting across datasets
Removing duplicate records
Eliminating spelling, naming conventions, or categorization inconsistencies
Validating data against rules and benchmarks.
Organizations risk basing their decisions on inaccurate or misleading information without proper data cleaning, leading to financial losses, incorrect insights, and operational inefficiencies.
Why is Data Cleaning Important?
Data is one of the most valuable assets for any business. However, raw data is often messy due to errors from human entry, system migrations, or external data imports. If left uncleaned, this dirty data can cause:
A. Poor Decision-Making
Unclean data leads to inaccurate reports, incorrect trends, and flawed insights.
Example: A retail company analyzing customer demographics might draw erroneous conclusions if duplicate records cause one customer to be counted multiple times.
B. Financial & Reputational Damage
Dirty data can lead to mispriced products, wrong customer segmentation, or incorrect invoices being sent.
Example: An eCommerce company might lose revenue by offering discounts to the wrong customer segment due to poor data categorization.
C. Decreased Productivity & Increased Manual Work
Without automated data cleaning, employees spend hours manually fixing errors.
Example: A data analyst may waste 40%–60% of their time correcting messy datasets instead of focusing on insights.
D. Compliance & Regulatory Risks
Many industries (e.g., healthcare, finance, legal) must maintain clean data for regulatory compliance.
Example: A financial institution might face penalties if incorrect customer data affects KYC (Know Your Customer) compliance.
By implementing AI-driven data cleaning tools, businesses can ensure that data is always accurate, consistent, and structured—enabling better decision-making, operational efficiency, and compliance.
Common Data Issues That Require Cleaning
Raw data is rarely perfect. It often contains errors, inconsistencies, and formatting issues that must be corrected before analysis. The most common issues include:
A. Missing Data (Incomplete Records) Problem
Gaps in datasets where essential values (e.g., customer emails, product prices, timestamps) are missing.
Example: A sales report's "Total Revenue" column is missing values, making it impossible to calculate accurate profits.
Solution: AI-powered tools can fill in missing values using machine learning predictions or statistical methods (e.g., median imputation).
B. Duplicate Data (Repeated Entries) Problem
The same customer, transaction, or product appears multiple times in a dataset, leading to overestimated counts.
Example: A customer appears in the CRM three times with slightly different name spellings (John Doe, Jon Doe, J. Doe), leading to inaccurate customer reports.
Solution: AI-driven de-duplication algorithms identify and merge duplicate records automatically.
C. Inconsistent Formatting Problem
Data is stored in different formats, making it hard to standardize and analyze.
Example: Dates appear in multiple formats (MM/DD/YYYY, DD/MM/YYYY, YYYY-MM-DD) across different regions, confusing time-sensitive analysis.
Solution: AI-powered data cleaning tools can automatically standardize date formats across the dataset.
D. Incorrect Data Entries (Typos, Spelling Errors, Mismatched Fields)
Problem: Errors caused by manual data entry mistakes or poorly formatted imports.
Example: A product catalog has inconsistent naming conventions: "Nike Air Max 2025," "nike_airmax_2025," and "Nike Air Max 25." This makes it impossible to track sales of the same product accurately.
Solution: AI-driven text standardization tools fix inconsistencies and align product names automatically.
E. Outdated or Irrelevant Data Problem
Datasets contain old or obsolete information.
Example: A customer database still contains records of users who haven't purchased in over 10 years, distorting marketing campaign results.
Solution: AI-powered tools can detect outdated records and suggest actions such as archiving or removal.
How AI is Transforming Data Cleaning in 2025
Traditionally, data cleaning was a manual, time-consuming process requiring hours of work from data analysts. However, AI-powered automation tools like Numerous have changed the game by making data cleaning faster, more innovative, and more efficient.
A. AI-Powered Automation Eliminates Repetitive Tasks
Instead of manually fixing errors, AI can automatically detect and correct missing values, identify duplicate entries, and intelligently merge them. It can also standardize formatting (dates, numbers, capitalization, etc.).
B. AI Predicts & Fills in Missing Data
Using machine learning, AI can Predict missing values based on existing patterns. Auto-fill incomplete records to maintain data integrity. Suggest corrections based on previous clean data.
C. AI Can Clean Data in Real-Time
With tools like Numerous, AI can continuously monitor and clean incoming data, ensuring that mistakes are fixed instantly instead of accumulating over time.
D. AI Integration in Spreadsheets Saves Time & Effort
AI-powered spreadsheet tools, like Numerous, enable users to clean data directly in Excel or Google Sheets using a simple prompt.
Instead of spending hours on formulas, users can type:
"Find and delete duplicate rows,"
"Standardize phone numbers to international format,"
"Identify and correct inconsistent product names."
Numerous then execute these tasks instantly, saving hours of manual work.
Related Reading
• Data Cleaning Process
• Data Cleaning Example
• How to Validate Data
• Data Validation Techniques
• Data Cleaning Best Practices
• Data Validation Best Practices
• Data Cleaning Example
How to Write AI Prompts for Data Cleaning (Step-by-Step Guide)

AI prompts are natural language commands or questions that inform an AI tool of precisely what to do. Users can describe their intent in plain language rather than relying on formulas, scripts, or manual inputs, and the AI automates the task.
For example, without AI, cleaning up a date format in a spreadsheet requires using a complex formula like =TEXT(A2, "YYYY-MM-DD"). This formula manually converts a date to a standardized format. With AI, you simply type: "Convert all dates in Column A to YYYY-MM-DD format." The AI automatically detects and applies the formatting to the entire column.
Best Practices for Writing Effective AI Prompts
To ensure AI understands and executes your request correctly, follow these best practices:
Be Clear and Specific
AI tools work best with precise instructions. Instead of saying, "Clean up my data," specify precisely what needs cleaning.
Good Example: "Find and remove duplicate email addresses in Column C while keeping the first occurrence."
Bad Example: "Remove duplicates." (Too vague—what column? Which duplicate should be kept?)
Use Action Words
AI responds well to clear, direct actions like convert, clean, find, remove, standardize, categorize, or format.
Good Example: "Find and replace all instances of 'N/A' in Column B with 'Not Available'."
Bad Example: "Change data." (It is too vague. What data needs changing? How should it be changed?)
Specify Columns or Data Ranges
AI works on entire datasets, so specifying columns or ranges prevents mistakes.
Good Example: "Standardize capitalization in Column D so that each word starts with a capital letter."
Bad Example: "Fix capitalization." (Fix where? Capitalization in what way?)
Provide Formatting Details
If formatting is required, specify the desired format.
Good Example: "Convert all dates in Column A to YYYY-MM-DD format."
Bad Example: "Fix the date format." (What format should it be?)
Define Conditions for Cleaning Tasks
If conditional logic applies, include it in the prompt.
Good Example: "Remove duplicate rows only if the values in Column B and Column C are identical."
Bad Example: "Remove some duplicates." (How should AI determine which to remove?)
Use Natural Language, But Avoid Unnecessary Words
AI can process natural language, but overly conversational prompts may reduce accuracy.
Good Example: "Identify and highlight all cells in Column E that contain numbers lower than 10."
Bad Example: "Hey AI, can you please take a look at Column E and tell me which ones have numbers under 10? Thanks!" (Too wordy—keep it structured.)
How to Structure AI Prompts for Data Cleaning
Basic Structure of an AI Prompt
To write a clear, effective prompt, follow this simple structure: Action + Data Range + Condition (if needed) + Formatting (if required)
Examples of Well-Structured AI Prompts
Handling Missing Data: "Fill all missing values in Column D using the column's median value." "Identify and highlight all empty cells in this dataset."
Detecting & Removing Duplicates: "Find and remove duplicate rows in Column C while keeping the first occurrence." "Merge duplicate customer names in Column A, keeping the most recent record."
Standardizing Formatting: "Ensure all email addresses in Column F are in lowercase." "Convert all text in Column B to title case (capitalize first letter of each word)."
Text Cleaning & Categorization: "Replace all instances of 'N/A' with 'Not Available' in Column G." "Classify customer reviews in Column H as 'Positive,' 'Neutral,' or 'Negative' based on sentiment analysis."
Outlier & Error Detection: "Flag all sales amounts in Column E that are above $10,000 as outliers." "Identify and correct any negative numbers in the 'Revenue' column."
Using AI Prompts in Spreadsheets (Google Sheets & Excel)
Step-by-Step Guide to Implement AI Prompts in Numerous (Google Sheets & Excel)
Open Google Sheets or Microsoft Excel. Enable Numerous AI (if installed as an add-on or extension). Type your AI prompt into the input box or command section. Example: "Find and delete duplicate product codes in Column B while keeping the first occurrence." Review the AI’s suggestions. Click 'Apply' to execute the data cleaning process automatically. This removes the need for formulas or scripts, allowing users to instantly clean, standardize, and organize data.
Related Reading
• Challenges of Data Cleaning
• Automated Data Validation
• AI Data Validation
• Customer Data Cleansing
• Benefits of Using AI for Data Cleaning
• Data Cleansing Strategy
• Data Cleaning Checklist
• AI Data Cleaning Tool
• Machine Learning Data Cleaning
• Challenges of AI Data Cleaning
• Data Cleaning Methods
25 Best AI Prompts for Data Cleaning
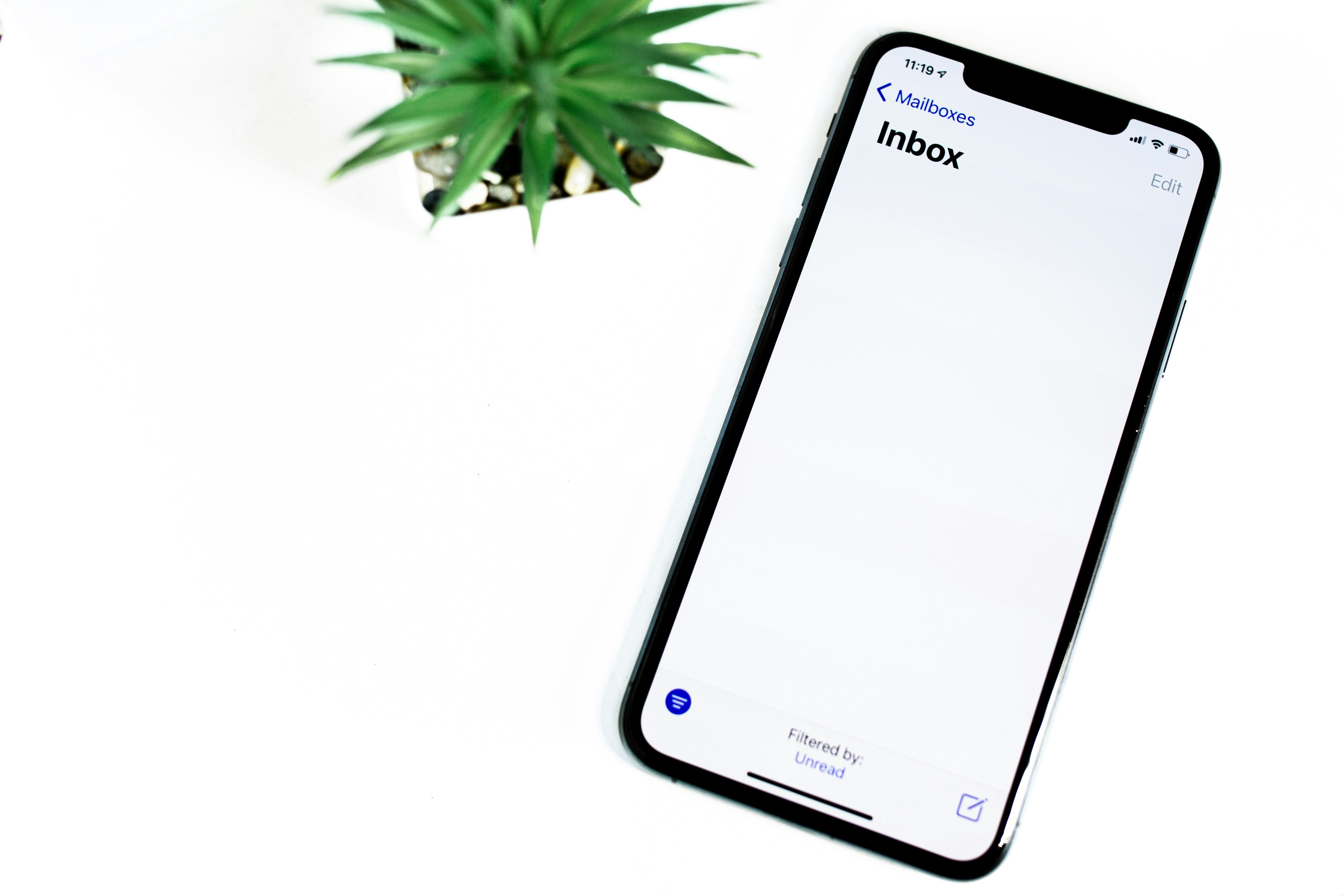
A. Handling Missing Data
Missing data can distort analysis and make datasets unreliable. The best AI prompts for handling missing data automatically identify, fill, or manage missing values.
"Identify and highlight all missing values in this dataset." This prompt uncovers all the gaps and helps you visualize where the problems are.
"Fill missing numerical values using the median of the column." This prompt fills gaps with the median value, often the best choice for avoiding bias in datasets with outliers.
"Fill missing numerical values using linear interpolation." This prompt fills gaps with values based on existing trends, ensuring a smooth transition between data points.
"Replace all missing text values with ‘Not Available’." This prompt standardizes missing text values to avoid confusion during analysis.
"Suggest the most likely values for missing entries based on existing patterns." This prompt uncovers existing patterns and helps you fill gaps with the most relevant values.
B. Detecting & Removing Duplicate Entries
Duplicate records inflate counts, cause errors in analysis, and mislead decision-making. The best AI prompts for cleaning duplicates efficiently detect and remove redundant records from datasets.
"Find and delete duplicate rows while keeping the first occurrence." This prompt uncovers exact duplicate entries and removes them automatically to streamline your dataset.
"Identify near-duplicate customer records and suggest merging strategies." This prompt uncovers variations of the same record that can mislead analysis and identifies ways to merge them.
"Highlight rows where the same email appears more than once." This prompt finds multiple occurrences of the same email address to help you investigate and remove redundant entries.
"Remove duplicate entries from this dataset while preserving the most recent record." This prompt identifies and deletes duplicate rows while ensuring you keep the latest version of the record.
"Find duplicate product names that have slight spelling variations." This prompt locates product names that are likely the same but have minor spelling differences, making them appear unique.
C. Standardizing Formatting Across Datasets
Inconsistent formatting makes data look unstructured and difficult to analyze. The best AI prompts for standardizing formatting across datasets ensure the data looks uniform.
"Convert all dates to the YYYY-MM-DD format." This prompt eliminates variations in date formatting by converting them all to the ISO standard, which is universally recognized.
“Ensure phone numbers follow international formatting (+CountryCode Number)." This prompt identifies and corrects any inconsistencies in phone number formatting so that all entries follow a standard format.
"Standardize all product names to Title Case formatting." This prompt corrects any inconsistencies in capitalizing product names and ensures they all follow Title Case formatting.
"Ensure all text entries in Column B follow sentence case." This prompt identifies text entries that are not in the proper case and corrects them automatically.
"Remove all leading and trailing spaces from text entries." This prompt eliminates any extra spaces in text entries that can cause errors during analysis.
D. Text Cleaning & Data Categorization
Unstructured text can cause inconsistencies in reporting and analytics. The best AI prompts for cleaning text data can structure, categorize, and organize text-based data.
"Standardize all company names by removing extra spaces and capitalization inconsistencies." This prompt cleans company names to ensure consistency for reporting and analysis.
"Identify and correct common spelling errors in this dataset." This prompt finds and fixes misspelled words in your dataset to improve overall quality.
“Classify customer reviews as Positive, Neutral, or Negative based on sentiment analysis." This prompt uses AI to understand the context of text entries and automatically categorize them into predefined groups.
"Extract only the domain names from a list of email addresses." This prompt removes all unnecessary text from your dataset and organizes the data into a more usable format.
"Identify and categorize product descriptions into predefined categories." This prompt analyzes product descriptions and uses existing rules to classify them for better organization.
E. Detecting Outliers & Errors in Data
Outliers can skew data insights and create misleading trends. The best AI prompts for detecting outliers automatically identify and correct such anomalies.
"Find all numerical values significantly higher or lower than the average." This prompt uncovers rogue data points that fall far outside the normal range and may need to be corrected or removed.
"Detect incorrect numerical values that do not match expected patterns (e.g., negative prices in a sales dataset)." This prompt identifies data points that are likely erroneous and need to be investigated before analysis.
"Flag inconsistent pricing for identical products." This prompt finds pricing variations for the same product that may result from data entry errors.
"Identify incorrectly formatted email addresses." This prompt locates email entries that do not follow standard formatting so you can fix them before analysis.
"Detect abnormal transaction amounts based on historical trends." This prompt analyzes past transaction data to establish a range of expected values and uncovers any recent entries that fall outside this norm.
Make Decisions At Scale Through AI With Numerous AI’s Spreadsheet AI Tool
AI prompts for data cleaning are designed to help users tackle the tedious, repetitive tasks accompanying large-scale data cleaning. This can include identifying and correcting errors within datasets and removing duplicate entries. Using these brilliant prompts, users can automate many of their data-cleaning tasks to help reduce human error and make the process far more efficient.
Numerous is an AI-Powered tool that enables content marketers, Ecommerce businesses, and more to do tasks many times over through AI, like writing SEO blog posts, generating hashtags, mass categorizing products with sentiment analysis and classification, and many more things by simply dragging down a cell in a spreadsheet. With a simple prompt, Numerous returns any spreadsheet function, simple or complex, within seconds.
The capabilities of Numerous are endless. It is versatile and can be used with Microsoft Excel and Google Sheets. Get started today with Numerous.ai so that you can make business decisions at scale using AI in both Google Sheets and Microsoft Excel. Learn more about how you can 10x your marketing efforts with Numerous’s ChatGPT for Spreadsheets tool.
Related Reading
• Talend Alternatives
• Informatica Alternatives
• Data Cleansing Tools
• AI vs Traditional Data Cleaning Methods
• Alteryx Alternative
• Data Validation Tools
© 2025 Numerous. All rights reserved.
© 2025 Numerous. All rights reserved.
© 2025 Numerous. All rights reserved.