In our rapidly evolving digital landscape, where information reigns supreme, harnessing the power of big data sentiment analysis has become an indispensable tool for businesses. By peering into the collective consciousness of online communities, we can uncover invaluable insights about consumer preferences, opinions, and emotions. Imagine having the ability to decode the sentiments behind every tweet, review, and comment, and using this knowledge to tailor marketing strategies with pinpoint accuracy. Welcome to the world of big data sentiment analysis.
Sentiment analysis marketing, or the process of extracting and analyzing sentiment from large volumes of data, has the potential to revolutionize the way companies connect with their target audience. Gone are the days of relying solely on intuition and guesswork. With the advent of advanced algorithms and machine learning techniques, businesses can now decipher the intricate nuances of human emotion on a scale never before possible.
By understanding the sentiment behind customer feedback, businesses can gain a deeper understanding of their audience, identify potential pain points, and adapt their marketing efforts accordingly. And in an era where customer satisfaction is paramount, this can mean the difference between thriving and merely surviving in today's competitive market. So, buckle up and join us on this thrilling journey into the realm of big data sentiment analysis, where the power of words and emotions hold the key to unlocking untapped business potential.
Table of Contents
How Sentiment Analysis Can Be Used To Identify and Mitigate Risks
Step-by-Step Guide On How To Do Big Data Sentiment Analysis With Numerous
How Sentiment Analysis Can Be Used For Social Media Monitoring
How Big Data Sentiment Analysis Can Help To Predict Customer Behavior
How To Integrate Sentiment Analysis With Other Data Analytics Techniques To Gain Deeper Insights
Make Decisions At Scale Through AI In Spreadsheets With Numerous
What Is Sentiment Analysis?

Sentiment analysis, also known as opinion mining, is a powerful technique that allows us to analyze and interpret the emotions, attitudes, and opinions expressed in large amounts of textual data. By leveraging the vast potential of big data, sentiment analysis enables us to gain valuable insights into public opinion, customer satisfaction, and brand perception.
The Importance of Sentiment Analysis in the Era of Big Data
Where information is generated at an unprecedented rate, sentiment analysis holds immense significance. Organizations across industries, from e-commerce and marketing to finance and politics, are leveraging this technique to understand their customers, gauge public sentiment, and make data-driven decisions.
By harnessing the power of big data, sentiment analysis enables businesses to process and analyze vast amounts of text data, such as social media posts, customer reviews, news articles, and customer feedback. This analysis allows them to identify trends, sentiment shifts, and emerging patterns, providing valuable insights that can shape their strategies and enhance their competitiveness.
How Does Sentiment Analysis Work?
Sentiment analysis involves several steps to accurately determine the sentiment expressed in text data. These steps include:
1. Text Preprocessing
The first step involves cleaning and preprocessing the text data to remove noise, punctuation, stopwords, and other irrelevant information. This process helps to ensure that the sentiment analysis model focuses on the meaningful content of the text.
2. Feature Extraction
In this step, relevant features are extracted from the preprocessed text. These features can include words, phrases, or even the context in which the sentiment is expressed. Advanced techniques like n-grams, word embeddings, and part-of-speech tagging can be used to capture the nuances of language.
3. Sentiment Classification
Once features are extracted, sentiment classification algorithms are employed to assign sentiment scores or labels to the text. These algorithms can range from simple rule-based approaches to more sophisticated machine learning models such as Support Vector Machines (SVM), Naive Bayes, or Recurrent Neural Networks (RNN).
4. Evaluation and Validation
After sentiment classification, the accuracy of the model is assessed using labeled data that indicates the correct sentiment for comparison. This step helps to refine the model and improve its performance.
Challenges and Limitations of Sentiment Analysis
While sentiment analysis has proven to be a valuable tool, it does come with certain challenges and limitations. Some of these include:
1. Contextual Understanding
Understanding the sentiment expressed in text often requires context comprehension, as words can have different meanings depending on the context in which they are used. This challenge is more prominent when dealing with sarcasm, irony, or ambiguity.
2. Language and Cultural Nuances
Sentiment analysis models need to account for language variations, slang words, and cultural nuances that may affect sentiment interpretation. Different languages and cultures may express sentiment differently, making it essential to consider these variations when building sentiment analysis models.
3. Domain-specific Sentiment
Sentiment analysis models may struggle to accurately interpret sentiment in domain-specific texts. Industries with specialized jargon, such as healthcare or legal fields, may require domain-specific sentiment lexicons or customized models for optimal results.
4. Subjectivity and Bias
Sentiment analysis is subjective by nature, as different individuals may interpret sentiment differently. Biases within the data used for training the models can lead to skewed results. It is crucial to continuously evaluate and address these biases to ensure fair and accurate sentiment analysis.
Sentiment analysis, fueled by big data, has revolutionized the way we understand and interpret human emotions and opinions expressed in textual data. This powerful technique enables businesses to make data-driven decisions, enhance customer experience, and stay ahead of the competition. While there are challenges and limitations to overcome, sentiment analysis continues to evolve, offering new possibilities for leveraging the vast amount of data generated in our digital world.
Related Reading
• Sentiment Analysis Using Product Review Data
• How Can Sentiment Analysis Be Used To Improve Customer Experience?
• Types Of Sentiment Analysis
• Sentiment Analysis Use Cases
• Sentiment Analysis In Healthcare
• Sentiment Analysis Customer Service
• Benefits Of Sentiment Analysis
• Sentiment Analysis Survey
• Voice Of Customer Sentiment Analysis
What Is Big Data Sentiment Analysis?

Businesses are constantly seeking ways to gain insights into customer opinions, emotions, and behaviors. One such method that has gained popularity is big data sentiment analysis. This cutting-edge technique leverages the power of artificial intelligence and machine learning algorithms to analyze a vast amount of data and uncover sentiment patterns, giving businesses a deeper understanding of customer perception and allowing them to make informed decisions.
Understanding Big Data Sentiment Analysis
Big data sentiment analysis, also known as opinion mining, refers to the process of automatically categorizing and analyzing emotions, attitudes, and opinions expressed in a large corpus of text data. This includes customer reviews, social media posts, news articles, and any other form of textual information available online. The goal is to determine whether the sentiment expressed in the text is positive, negative, or neutral.
Traditional sentiment analysis methods typically involve manually creating a list of predefined sentiment words or phrases and classifying text based on the presence of these words. While this approach can be useful for smaller datasets, it falls short when dealing with large volumes of unstructured text data. That's where big data sentiment analysis comes into play, offering several key advantages:
1. Scale and Speed
Traditional sentiment analysis methods struggle to cope with the sheer scale of big data. Big data sentiment analysis, on the other hand, is designed to handle massive amounts of data efficiently. By employing distributed computing frameworks and parallel processing techniques, it can process and analyze vast quantities of text data in real-time, providing businesses with timely insights.
2. Contextual Understanding
Big data sentiment analysis algorithms go beyond simplistic keyword matching. They employ advanced natural language processing techniques to understand the context, sarcasm, irony, and idiomatic expressions present in the text. This contextual understanding enables more accurate sentiment classification, ensuring that the true meaning behind the words is captured.
3. Multilingual Analysis
Businesses operate in multiple languages, and understanding sentiment across different languages is crucial. Traditional sentiment analysis methods often struggle to handle multilingual data, but big data sentiment analysis can effortlessly analyze sentiment in various languages. This capability allows businesses to gain a comprehensive understanding of sentiment across diverse markets and demographics.
4. Real-time Insights
The ability to extract sentiment in real-time is a significant advantage of big data sentiment analysis. By continuously monitoring social media platforms, online forums, and other sources of customer feedback, businesses can promptly identify emerging trends, potential PR crises, and customer satisfaction levels. This real-time insight enables businesses to respond swiftly to customer concerns and adapt their strategies accordingly.
5. Domain Adaptation
One challenge in sentiment analysis is adapting the algorithms to specific industries or domains. Traditional sentiment analysis methods often require extensive manual customization to perform well in a particular domain. In contrast, big data sentiment analysis algorithms can be trained on large domain-specific datasets, allowing them to adapt and perform effectively across different industries with minimal manual intervention.
Big data sentiment analysis revolutionizes the way businesses understand customer sentiment. By harnessing the power of advanced technologies and scalable processing frameworks, it provides businesses with real-time, actionable insights into customer opinions and emotions, enabling them to make data-driven decisions and enhance customer satisfaction. With its ability to handle massive volumes of data, understand context, and analyze sentiment in multiple languages, big data sentiment analysis is a game-changer in the field of sentiment analysis.
Why Is Sentiment Analysis Important?

Understanding the sentiments and emotions of customers, stakeholders, and the general public is crucial for businesses and decision-makers. Sentiment analysis, also known as opinion mining, is a valuable tool that helps them gain insights into how people perceive their brand, products, and services. By analyzing big data, which includes social media posts, customer reviews, and online discussions, businesses can uncover valuable information that enables them to make informed decisions and improve their strategies. Let's explore the reasons why sentiment analysis is vital for businesses and decision-makers.
1. Customer Satisfaction and Retention
One of the primary reasons for utilizing sentiment analysis is to assess customer satisfaction levels. By analyzing customer feedback and sentiment, businesses can gauge how well their products or services meet customer needs and expectations. Positive sentiments indicate satisfied customers who are likely to become loyal advocates, while negative sentiments highlight areas for improvement. This insight helps businesses address customer concerns promptly, enhance their offerings, and ultimately retain satisfied customers.
2. Brand Reputation Management
Sentiment analysis plays a crucial role in brand reputation management. Businesses can monitor online conversations about their brand in real-time and detect any negative sentiment or potential issues. By promptly addressing these concerns, businesses can mitigate reputational damage, maintain a positive brand image, and build trust with their customers and stakeholders.
3. Product Development and Innovation
Sentiment analysis enables businesses to gain valuable insights into customer preferences and expectations. By analyzing sentiment around specific product features or ideas, decision makers can identify trends, preferences, and gaps in the market. This information is invaluable in guiding product development and innovation efforts, ensuring that businesses can create offerings that resonate with their target audience.
4. Competitive Analysis
Sentiment analysis provides a competitive advantage by allowing businesses to monitor the sentiment around their competitors. By understanding how customers perceive competing brands, businesses can identify areas of strength and weakness. This information helps decision-makers develop strategies to differentiate their brand, improve their offerings, and gain a competitive edge in the market.
5. Crisis Management and Risk Mitigation
In today's digital age, negative sentiment can quickly escalate and impact a brand's reputation. Sentiment analysis allows businesses to detect and address negative sentiment in real-time, helping them mitigate potential crises. By monitoring sentiment, decision makers can identify emerging issues, assess their impact, and take proactive measures to manage risks effectively.
6. Customer Feedback Analysis
Sentiment analysis helps businesses analyze customer feedback at scale. Instead of manually reviewing each piece of feedback, sentiment analysis algorithms can automatically categorize and analyze customer sentiment. This saves time and resources, allowing decision makers to focus on strategic initiatives rather than spending hours deciphering feedback.
Sentiment analysis is a powerful tool that provides businesses and decision makers with valuable insights. By analyzing big data and understanding customer sentiment, businesses can improve customer satisfaction, manage their brand reputation, guide product development, stay ahead of the competition, and effectively manage crises. Leveraging sentiment analysis allows businesses to make data-driven decisions and enhance their strategies, ultimately leading to improved business outcomes.
How Sentiment Analysis Can Be Used To Identify and Mitigate Risks

In today's interconnected world, businesses face numerous risks and potential crises that can impact their reputation, customer trust, and ultimately their bottom line. One way to proactively address these challenges is by utilizing big data sentiment analysis. This powerful tool can help businesses identify and mitigate potential risks and crises by analyzing public sentiment towards their brand, products, and services. We will explore how sentiment analysis can be effectively used for risk management and crisis prevention.
1. Enhancing Brand Reputation Monitoring
Building a strong brand reputation is crucial for any business. Sentiment analysis allows businesses to monitor public sentiment towards their brand in real-time. By analyzing social media posts, customer reviews, and online discussions, businesses can gain valuable insights into how their brand is being perceived by the public. This information can help identify potential risks to their reputation, such as negative sentiment towards a specific product or service, customer complaints, or emerging trends that may impact their brand image.
2. Early Detection of Negative Trends
Sentiment analysis can help businesses identify negative trends and potential crises before they escalate. By monitoring sentiment across various channels, businesses can detect shifts in public opinion and identify emerging issues. For example, if there is a sudden increase in negative sentiment towards a specific feature of a product, businesses can take immediate action to address the issue before it becomes a full-blown crisis. This early detection allows businesses to be proactive in their response and mitigate potential risks.
3. Crisis Response and Damage Control
In the event of a crisis, sentiment analysis can play a crucial role in crafting an effective crisis response strategy. By analyzing sentiment and identifying the key concerns and grievances of the public, businesses can tailor their messaging and actions to address these issues directly. This targeted approach can help rebuild trust and mitigate the negative impact of the crisis on the brand's reputation. Sentiment analysis also allows businesses to monitor the effectiveness of their crisis response in real-time, enabling them to make necessary adjustments and improvements as needed.
4. Competitive Analysis
Sentiment analysis can also be utilized for competitive analysis, helping businesses stay ahead of their rivals. By analyzing sentiment towards competitors' brands, products, and services, businesses can gain insights into their strengths and weaknesses. This information can be used to identify potential opportunities for differentiation and improvement. Sentiment analysis can help businesses identify potential risks or crises that their competitors may be facing, allowing them to learn from their mistakes and proactively address similar issues.
Big data sentiment analysis is a powerful tool that can help businesses identify and mitigate potential risks and crises. By effectively monitoring public sentiment, businesses can enhance their brand reputation, detect negative trends early, respond to crises effectively, and stay ahead of their competitors. Incorporating sentiment analysis into risk management strategies can greatly improve a business's ability to navigate the ever-changing landscape of public opinion and ensure long-term success.
Step-by-Step Guide On How To Do Big Data Sentiment Analysis With Numerous
Watch this guide on how to use ChatGPT for classification and sentiment analysis in Google Sheets and Microsoft Excel with Numerous AI:
Google Sheets Guides
Microsoft Excel Guide
How Sentiment Analysis Can Be Used For Social Media Monitoring

Social media has become a powerful platform for individuals and businesses to connect and interact with one another. With the vast amount of data generated on social media platforms, it can be challenging for brands to keep track of what people are saying about their products or services. This is where sentiment analysis comes in.
Sentiment analysis, also known as opinion mining, is a process of analyzing and categorizing text data to determine the sentiment expressed within it. It involves using natural language processing (NLP) techniques to identify and classify the sentiment of social media posts, comments, reviews, and other forms of online content.
1. Tracking Brand Sentiment
Sentiment analysis allows brands to monitor the sentiment associated with their brand on social media platforms. By analyzing the sentiment of social media mentions, brands can identify positive and negative sentiments towards their brand and track how these sentiments change over time. This information can help them gauge customer satisfaction, identify potential issues, and take proactive measures to address any negative sentiment.
2. Identifying Customer Sentiment
With sentiment analysis, brands can obtain a deeper understanding of customer sentiment towards specific products or services. By analyzing social media conversations and reviews, brands can identify recurring themes, sentiment trends, and common pain points. This information can help them identify areas for improvement, develop better products or services, and tailor their marketing messages to resonate with their target audience.
3. Crisis Management
Sentiment analysis is an invaluable tool for brand reputation management, especially during times of crisis. By monitoring social media sentiment in real-time, brands can quickly identify and respond to negative sentiment that may arise due to a product issue, a PR crisis, or any other unforeseen event. By addressing these issues promptly and transparently, brands can mitigate reputational damage and maintain the trust and loyalty of their customers.
4. Competitive Analysis
Sentiment analysis can also be leveraged for competitive analysis. By analyzing the sentiment associated with competitors' brands, products, or services, brands can gain insights into their market positioning, strengths, weaknesses, and customer perceptions. This information can help them identify opportunities for differentiation, benchmark their own brand sentiment against competitors, and refine their marketing strategies accordingly.
5. Influencer Marketing
Sentiment analysis can assist brands in identifying relevant influencers and measuring the impact of influencer marketing campaigns. By analyzing the sentiment of social media posts and comments related to influencers, brands can assess the authenticity and effectiveness of influencer partnerships. This information can help them identify the most influential voices within their target audience and allocate resources to the most impactful influencer collaborations.
Sentiment analysis plays a crucial role in social media monitoring and brand reputation management. By leveraging this powerful tool, brands can gain valuable insights into customer sentiment, track brand perception, manage crises effectively, analyze competition, and optimize influencer marketing campaigns. By harnessing the power of big data sentiment analysis, brands can make data-driven decisions that drive customer satisfaction, loyalty, and ultimately, business success.
How Big Data Sentiment Analysis Can Help To Predict Customer Behavior

Understanding customer behavior and preferences is crucial for businesses to stay competitive and meet the evolving needs of their target audience. Big data sentiment analysis can play a significant role in predicting customer behavior and preferences by providing valuable insights into their emotions, opinions, and attitudes toward products or services. We will explore how big data sentiment analysis can help businesses gain a deeper understanding of their customers and make informed decisions to enhance customer satisfaction and drive business growth.
1. Unearthing Customer Sentiment: The Power of Big Data
Big data sentiment analysis harnesses the power of vast amounts of data to uncover customer sentiment. It involves collecting and analyzing data from various sources, such as social media, customer feedback, online reviews, and surveys, to identify patterns and trends in customer opinions. By employing advanced natural language processing (NLP) techniques, sentiment analysis algorithms can categorize customer sentiment as positive, negative, or neutral, providing a holistic view of customer perception.
2. Identifying Customer Preferences and Needs
By analyzing customer sentiment, businesses can gain insights into customer preferences and needs. Sentiment analysis can reveal which features or aspects of a product or service customers appreciate or dislike the most.
For example, by tracking sentiment around specific product features mentioned in customer reviews, businesses can identify areas of improvement or innovation. This information can guide decision-making processes, leading to the development of products or services that align better with customer expectations.
3. Enhancing Customer Experience
Big data sentiment analysis can help businesses improve the overall customer experience. By identifying negative sentiment or dissatisfaction, companies can proactively address customer concerns, resolve issues, and take prompt action to rectify any shortcomings. Timely response to customer feedback can demonstrate a commitment to customer satisfaction, fostering loyalty and positive brand perception.
4. Personalized Marketing and Recommendations
Sentiment analysis can enable businesses to personalize marketing campaigns and recommendations based on customer sentiment. By understanding customer preferences, businesses can tailor their messaging and content to resonate better with their target audience.
For instance, sentiment analysis can identify customers who have expressed positive sentiment towards a particular product and then target them with personalized offers or recommendations, increasing the likelihood of conversions.
5. Forecasting Customer Behavior and Trends
Sentiment analysis can also help in forecasting customer behavior and predicting future trends. By analyzing sentiment patterns over time, businesses can identify emerging trends, anticipate customer demands, and adapt their strategies accordingly.
For example, if sentiment analysis reveals a growing interest in eco-friendly products, businesses can invest in sustainable initiatives or launch environmentally friendly products to capitalize on this trend.
6. Competitor Analysis and Market Insights
Big data sentiment analysis can provide valuable insights into competitors and the overall market landscape. By analyzing customer sentiment towards competing products or brands, businesses can identify strengths and weaknesses, benchmark their performance, and gain a competitive edge. This information can guide strategic decision-making and help businesses identify market opportunities or potential threats.
Big data sentiment analysis offers businesses a powerful tool to predict customer behavior and preferences. By analyzing customer sentiment, businesses can unearth valuable insights that can drive decision-making, enhance customer experience, personalize marketing efforts, forecast trends, and gain a competitive advantage. Leveraging the power of big data sentiment analysis can empower businesses to meet customer expectations more effectively and stay ahead in today's dynamic market.
Using Sentiment Analysis for Market Research
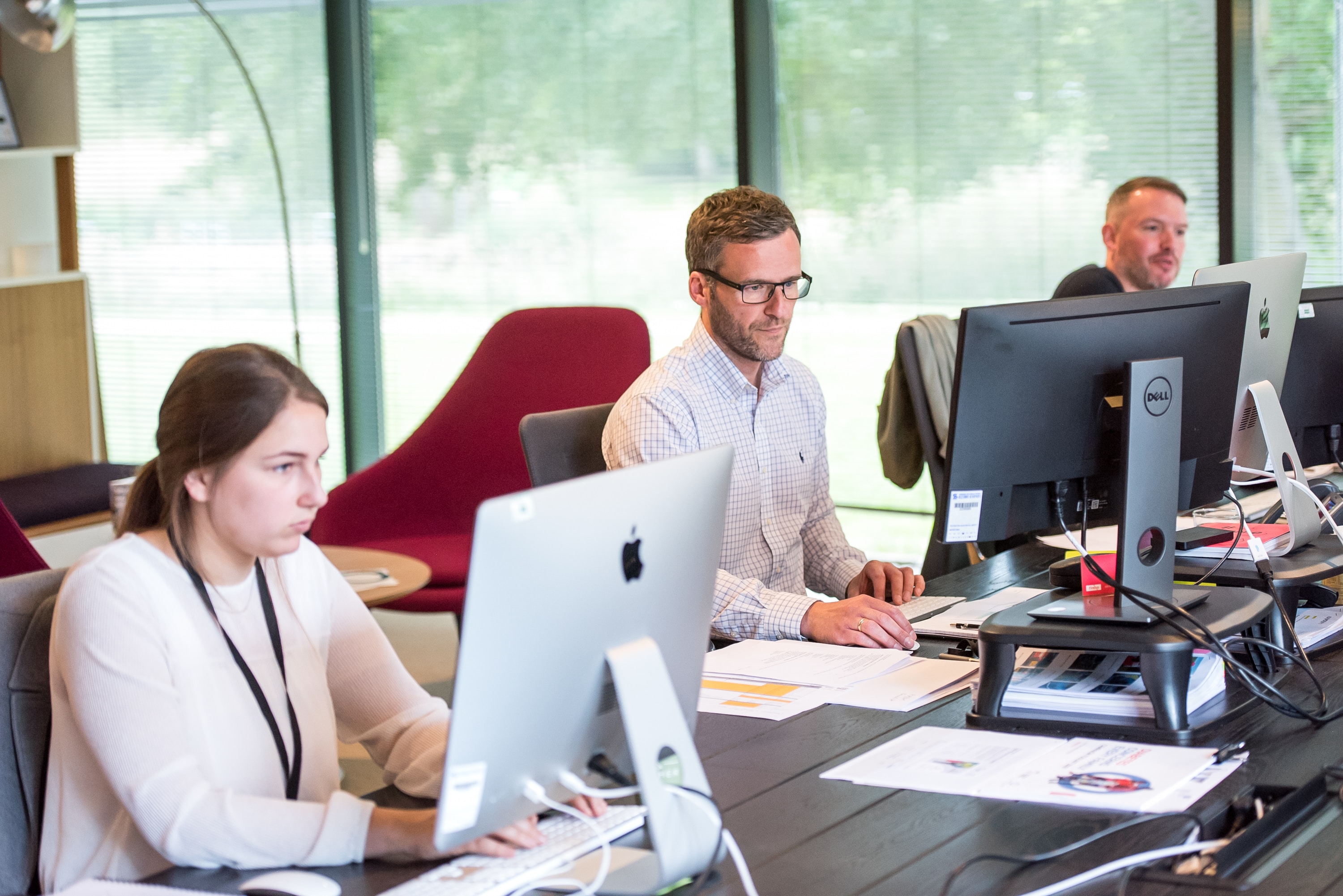
Businesses are constantly seeking new ways to gain a competitive edge. One powerful tool that can provide valuable insights is big data sentiment analysis. By analyzing large volumes of data from various sources, such as social media, customer reviews, and online forums, businesses can uncover valuable information about customer sentiment and preferences. This information can then be utilized in market research and competitor analysis to inform strategic decision-making and drive business growth.
Understanding Customer Sentiment
One of the key benefits of big data sentiment analysis is its ability to uncover the true feelings and opinions of customers. Traditional market research methods, such as surveys and focus groups, often suffer from response bias or limited sample sizes. Big data sentiment analysis, on the other hand, allows businesses to tap into a vast amount of unfiltered customer feedback.
By analyzing the sentiment expressed in customer reviews, social media posts, and online discussions, businesses can gain a deeper understanding of customer satisfaction, preferences, and pain points. This information can be used to identify trends and patterns, identify areas for improvement, and develop targeted marketing strategies.
Identifying Market Trends and Opportunities
In addition to understanding customer sentiment, big data sentiment analysis can help uncover emerging market trends and opportunities. By analyzing large volumes of data from various sources, businesses can identify patterns and correlations that may not be immediately apparent.
For example, by analyzing social media conversations, businesses can identify emerging trends and topics of interest to their target audience. This can help them stay ahead of the curve and develop innovative products or services that cater to changing customer needs.
Competitor Analysis
Big data sentiment analysis also plays a crucial role in competitor analysis. By monitoring and analyzing customer sentiment towards competitors, businesses can gain valuable insights into their strengths, weaknesses, and market positioning.
Strategic Insights
For example, by analyzing customer reviews of competing products or services, businesses can identify areas where competitors are excelling or falling short. This information can be used to benchmark against competitors, identify opportunities for differentiation, and develop targeted marketing campaigns.
Market Expansion Opportunities
By analyzing customer sentiment towards competitors, businesses can identify gaps in the market and potential areas for expansion. By understanding what customers are looking for and where competitors are falling short, businesses can develop new products or services that meet unmet customer needs.
Big data sentiment analysis is a powerful tool that can enhance market research and competitor analysis. By analyzing large volumes of data from various sources, businesses can gain insights into customer sentiment, identify market trends, and uncover opportunities for growth. By leveraging these insights, businesses can make informed decisions and stay ahead of the competition.
Potential Applications of Big Data Sentiment Analysis

Big data sentiment analysis is a powerful tool that allows businesses to gain valuable insights into customer sentiment and make data-driven decisions. By analyzing large volumes of data and extracting sentiment-related information, companies can better understand customer preferences, identify trends, and improve their products and services. The potential applications of big data sentiment analysis are wide-ranging and can greatly benefit industries across the board.
1. Customer Experience Enhancement
One of the most significant applications of big data sentiment analysis is in enhancing the customer experience. By analyzing customer feedback, reviews, and social media posts, businesses can identify patterns and trends in customer sentiment. This information can help companies improve their products, services, and overall customer satisfaction. For example, a hotel chain can analyze customer reviews on various platforms to identify common complaints and take corrective measures to enhance their guests' experience.
2. Brand Reputation Management
Big data sentiment analysis is instrumental in brand reputation management. By monitoring and analyzing social media conversations, online reviews, and news articles, companies can promptly identify any negative sentiment surrounding their brand. This allows them to address issues and protect their reputation in real-time. For instance, a retail company can detect negative sentiment on social media about a faulty product and take immediate action to rectify the situation, demonstrating its commitment to customer satisfaction.
3. Market Research and Competitor Analysis
In the field of market research, big data sentiment analysis can provide valuable insights into consumer preferences and opinions. By analyzing social media posts, online forums, and customer reviews, businesses can identify emerging trends, understand customer sentiments towards specific products or services, and gain a competitive advantage. For example, an automobile manufacturer can analyze sentiment data to understand customer perceptions of electric vehicles and develop marketing strategies accordingly.
4. Financial and Investment Analysis
Big data sentiment analysis can also be applied in the financial and investment industry. By analyzing news articles, social media, and financial reports, analysts can gauge market sentiment and make informed investment decisions. Sentiment analysis can help identify market trends, investor sentiment towards specific stocks, or even predict potential market movements. This information can be invaluable for investors and financial institutions in managing risks and maximizing returns.
5. Healthcare and Public Health
Sentiment analysis of patient feedback and online health forums can be utilized in the healthcare industry to improve patient care and public health. By analyzing patient reviews and experiences, healthcare providers can identify areas of improvement and enhance the quality of care. Sentiment analysis can also be used to monitor public sentiment towards health-related issues, such as vaccine acceptance or reactions to new medical treatments.
The applications of big data sentiment analysis are far-reaching and can benefit industries of all types. From enhancing customer experiences to managing brand reputation, from market research to financial analysis, and even in healthcare, sentiment analysis provides valuable insights that can guide decision-making and improve overall performance. By harnessing the power of big data sentiment analysis, businesses can stay ahead of the competition, adapt to changing market trends, and ultimately provide better products and services to their customers.
How To Integrate Sentiment Analysis With Other Data Analytics Techniques To Gain Deeper Insights

Sentiment analysis has emerged as a powerful tool for extracting valuable insights from vast amounts of unstructured textual data. By analyzing the sentiment behind customer reviews, social media posts, and other forms of online content, organizations can gain a deeper understanding of customer opinions and preferences. To fully leverage the potential of sentiment analysis, it is crucial to integrate it with other data analytics techniques. This integration allows for a more comprehensive and nuanced analysis, leading to even deeper insights.
1. Combining Sentiment Analysis with Text Classification
Integrating sentiment analysis with text classification techniques can provide a more nuanced understanding of customer feedback. Text classification involves categorizing text into predefined categories based on its content. By combining sentiment analysis with text classification, organizations can not only determine the sentiment behind customer feedback but also classify the feedback based on other relevant attributes.
For example, sentiment analysis can be combined with product classification to determine the sentiment of customer reviews for specific product categories. This integration enables organizations to identify sentiment trends specific to different product lines or features, allowing them to make more targeted improvements.
2. Incorporating Sentiment Analysis into Predictive Analytics
Predictive analytics leverages historical data to make predictions about future outcomes. By integrating sentiment analysis into predictive analytics models, organizations can gain valuable insights into how customer sentiment impacts future behavior.
For example, sentiment analysis can be used to assess customer sentiment towards a new product launch. By incorporating sentiment analysis results into predictive models, organizations can make more accurate predictions about the potential success of the product and adjust their marketing strategies accordingly. This integration allows organizations to proactively address potential issues and optimize their decision-making processes.
3. Integrating Sentiment Analysis with Network Analysis
Network analysis focuses on understanding the relationships and connections between individuals or entities. By integrating sentiment analysis with network analysis, organizations can uncover hidden patterns and relationships that impact sentiment.
For instance, social network analysis can be combined with sentiment analysis to identify influential individuals or groups that drive sentiment within a particular community. This integration can help organizations identify key opinion leaders and tailor their marketing strategies to effectively target and engage these influencers.
4. Fusion of Sentiment Analysis with Visual Analytics
Visual analytics enables the exploration and visualization of complex data sets to uncover patterns and trends. By fusing sentiment analysis with visual analytics, organizations can gain a visual representation of sentiment patterns and trends.
Visualizing sentiment analysis results can help identify clusters of positive or negative sentiment, allowing organizations to quickly understand the overall sentiment landscape. Visual analytics can help identify correlations between sentiment and other data variables, enabling organizations to gain deeper insights into factors influencing sentiment.
5. Integration of Sentiment Analysis with Time Series Analysis
Time series analysis focuses on analyzing data that changes over time. By integrating sentiment analysis with time series analysis, organizations can monitor and analyze changes in sentiment over a specific period. This integration allows organizations to identify sentiment trends, seasonality, or other time-based patterns.
For example, sentiment analysis combined with time series analysis can help organizations understand how sentiment towards their brand fluctuates throughout the year, enabling them to optimize their marketing campaigns accordingly.
By integrating sentiment analysis with other data analytics techniques such as text classification, predictive analytics, network analysis, visual analytics, and time series analysis, organizations can gain a more comprehensive understanding of customer sentiment and its impact on their business. This holistic approach to data analysis enables organizations to make informed decisions, improve customer satisfaction, and drive business growth.
Related Reading
• Sentiment Analysis Example
• Sentiment Analysis Social Media
• Brand Sentiment Analysis
• Online Sentiment Analysis
• How To Do Sentiment Analysis
• Sentiment Analysis Twitter
• Reddit Sentiment Analysis
• Sentiment Analysis Application
• Sentiment Analysis Of Reviews
• Lexicon Based Sentiment Analysis
• Sentiment Analysis Finance
• Sentiment Analysis Steps
• Amazon Review Sentiment Analysis
Make Decisions At Scale Through AI In Spreadsheets With Numerous
In the ever-evolving world of digital marketing, content is king. But creating high-quality, engaging, and optimized content can be a time-consuming and labor-intensive task. That's where Numerous.ai comes in. This AI-powered tool is a game-changer for content marketers, ecommerce businesses, and more, offering a wide range of capabilities that can revolutionize your content marketing strategy.
AI-Powered Content Generation
One of the most impressive features of Numerous.ai is its ability to generate content using artificial intelligence. With just a simple prompt, Numerous.ai can write SEO blog posts, generate hashtags, and even categorize products using sentiment analysis and classification. This means you can create high-quality, optimized content at scale, saving you time and effort.
Mass Categorization with Sentiment Analysis
Sentiment analysis is a valuable tool for understanding the emotions and opinions expressed in large amounts of text data. With Numerous.ai, you can use sentiment analysis to automatically categorize products based on customer reviews, social media mentions, and other sources of customer feedback. This allows you to quickly identify trends, evaluate customer satisfaction, and make data-driven decisions to improve your products and services.
Seamless Integration with Spreadsheets
Numerous.ai is designed to seamlessly integrate with Microsoft Excel and Google Sheets, making it accessible and easy to use for anyone familiar with these popular spreadsheet programs. With just a few clicks, you can access the power of AI and leverage the capabilities of Numerous.ai within your existing workflows.
Make Data-Driven Decisions at Scale
The capabilities of Numerous.ai are truly endless. Whether you're a content marketer looking to generate optimized content, an ecommerce business wanting to categorize products, or a business professional needing to perform complex spreadsheet functions, Numerous.ai has you covered. By harnessing the power of AI, you can make data-driven decisions at scale and unlock new opportunities for growth and success.
Get Started with Numerous.ai Today
Don't let manual tasks and time-consuming processes hold you back. Embrace the power of AI and revolutionize your content marketing strategy with Numerous.ai. With its versatile capabilities, seamless integration with spreadsheets, and ability to make business decisions at scale, Numerous.ai is a must-have tool for any modern marketer or business professional. Get started with Numerous.ai today and take your content marketing to new heights.
Related Reading
• Sentiment Analysis Api
• Sentiment Analysis Software
• Ai Sentiment Analysis
• Sentiment Analysis In Excel
• Online Sentiment Analysis Tool
• Gpt3 Sentiment Analysis
• Sentiment Analysis Google Sheets