In the ever-evolving landscape of sentiment analysis marketing, understanding the different types of sentiment analysis is crucial for businesses aiming to make data-driven decisions. By diving deep into the realm of sentiment analysis, companies can unlock invaluable insights into customer opinions, preferences, and emotions. From basic approaches to more advanced methodologies, the world of sentiment analysis is as vast as it is fascinating. So, let's embark on an exploration of the various types of sentiment analysis, uncovering the secrets that lie beneath the surface.
As we delve into the realm of types of sentiment analysis, we will unravel the intricacies of this powerful tool. From the simple polarity-based analysis, where sentiments are classified into positive, negative, or neutral, to the more nuanced aspect-based sentiment analysis, which allows one to discern emotions towards specific entities within a text, there are countless dimensions to explore. But sentiment analysis goes beyond mere classifications; it unlocks the ability to comprehend the depth and complexity of human emotions, empowering businesses to understand their customers on a deeper level. So buckle up as we embark on this journey to unravel the mysteries of sentiment analysis and discover how it can transform the way businesses connect with their audience.
Table of Contents
Common Challenges With Sentiment Analysis & How To Overcome Them
Step-by-Step Guide On How To Do Sentiment Analysis With Numerous
Key Success Factors When Implementing Sentiment Analysis To Make More Money
How Sentiment Analysis Can Be Combined With Other Analytics Techniques
Make Decisions At Scale Through AI In Spreadsheets With Numerous
What Is Sentiment Analysis?

Businesses are constantly searching for ways to gain a competitive edge. One powerful tool that is often overlooked is sentiment analysis. But what exactly is sentiment analysis, and why is it important for businesses?
Sentiment analysis, also known as opinion mining, is the process of extracting and analyzing emotions, attitudes, and opinions from textual data. It involves using natural language processing (NLP) techniques and machine learning algorithms to determine the sentiment expressed in a piece of text, such as a customer review, social media post, or survey response.
The Importance of Sentiment Analysis for Businesses
1. Customer Insights
Understanding the sentiment of customers is crucial for businesses to improve their products or services. Sentiment analysis allows companies to gain valuable insights into customer opinions, preferences, and satisfaction levels. By analyzing customer feedback, businesses can identify areas for improvement and make data-driven decisions to enhance customer experience.
2. Brand Monitoring
In the age of social media, brands need to be aware of what people are saying about them online. Sentiment analysis provides a way to monitor brand sentiment, helping companies stay on top of public perception and address any negative sentiment before it escalates. By identifying patterns and trends in customer sentiment, businesses can proactively manage their brand image and reputation.
3. Competitor Analysis
Sentiment analysis can also be used to gain a competitive advantage by analyzing the sentiment surrounding competitors. By understanding how customers feel about competitors' products or services, businesses can identify gaps in the market and develop strategies to differentiate themselves. This allows companies to stay one step ahead in the ever-evolving business landscape.
4. Crisis Management
When a crisis strikes, businesses need to act swiftly and effectively to mitigate the damage. Sentiment analysis can help companies monitor public sentiment during a crisis, allowing them to respond in a timely manner and address concerns before they spiral out of control. By understanding the sentiments of affected stakeholders, businesses can tailor their communication and take appropriate actions to rebuild trust.
5. Marketing Campaign Optimization
Sentiment analysis can provide valuable insights for marketing campaigns. By analyzing customer feedback and sentiment towards past campaigns, businesses can refine their marketing strategies and messaging to resonate with their target audience. This allows companies to create more impactful and engaging campaigns, leading to higher customer satisfaction and increased brand loyalty.
Sentiment analysis is a powerful tool that enables businesses to tap into the emotions and opinions of their customers. By understanding sentiment, companies can gain valuable insights, monitor brand perception, analyze competitors, manage crises, and optimize marketing campaigns. Incorporating sentiment analysis into business strategies allows companies to make data-driven decisions and stay ahead in today's competitive marketplace.
Related Reading
• How Can Sentiment Analysis Be Used To Improve Customer Experience?
• Sentiment Analysis Using Product Review Data
• Sentiment Analysis Use Cases
• Sentiment Analysis In Healthcare
• Sentiment Analysis Customer Service
• Big Data Sentiment Analysis
• Benefits Of Sentiment Analysis
• Sentiment Analysis Survey
• Voice Of Customer Sentiment Analysis
10 Types of Sentiment Analysis for Ecommerce

1. Tracking Customer Satisfaction
Sentiment analysis in ecommerce allows businesses to track customer satisfaction by analyzing feedback, reviews, and ratings. This helps businesses understand how customers perceive their products or services and identify areas for improvement. By monitoring sentiment, ecommerce businesses can gauge customer satisfaction levels and make informed decisions to enhance their offerings.
2. Assessing Product Reviews
Ecommerce businesses can benefit from sentiment analysis by assessing product reviews. By analyzing the sentiment expressed in reviews, businesses can identify positive and negative aspects of their products, as well as customer preferences and pain points. This information can guide product development and marketing strategies, ultimately leading to improved customer satisfaction and increased sales.
3. Understanding Brand Perception
Sentiment analysis can provide valuable insights into how customers perceive a brand. By analyzing social media posts, customer reviews, and online discussions, ecommerce businesses can gain an understanding of their brand reputation. This allows them to assess if their brand is being positively or negatively portrayed and take appropriate actions to shape public perception.
4. Identifying Customer Sentiment on Social Media
Social media platforms serve as a treasure trove of customer sentiment data. Ecommerce businesses can leverage sentiment analysis to monitor customer sentiment on social media. By analyzing tweets, comments, and posts, businesses can identify trends, understand customer perceptions, and engage with customers in a timely manner to address any concerns or issues.
5. Predicting Customer Churn
Sentiment analysis can be used to predict customer churn in ecommerce. By analyzing customer feedback and sentiment, businesses can identify signs of dissatisfaction or disengagement. This allows them to proactively take measures to retain customers, such as offering personalized discounts, addressing concerns, or improving customer service.
6. Optimizing Product Recommendations
E-commerce businesses can use sentiment analysis to optimize their product recommendation algorithms. By analyzing customer feedback and sentiment, businesses can better understand customer preferences and tailor their recommendations accordingly. This personalization can lead to improved customer satisfaction and increased conversion rates.
7. Monitoring Competitor Sentiment
Understanding competitor sentiment is crucial for ecommerce businesses. Sentiment analysis can help businesses monitor how customers perceive their competitors and identify areas where they can gain a competitive advantage. By analyzing customer reviews and social media conversations, businesses can gain insights into competitor strengths and weaknesses and adjust their strategies accordingly.
8. Analyzing Customer Support Interactions
Sentiment analysis can be applied to customer support interactions to assess customer satisfaction and identify areas for improvement. By analyzing chat logs, emails, and call recordings, ecommerce businesses can gauge the sentiment of customer interactions with support agents. This allows them to identify training needs, improve response times, and provide a better overall customer experience.
9. Assessing Advertising Effectiveness
Ecommerce businesses can use sentiment analysis to assess the effectiveness of their advertising campaigns. By analyzing customer sentiment expressed in online discussions and social media posts related to the advertising campaigns, businesses can gauge customer reactions and adjust their marketing strategies accordingly. This can help optimize advertising spend and maximize the impact of marketing efforts.
10. Detecting Fraudulent Reviews
Sentiment analysis can help ecommerce businesses detect fraudulent reviews. By analyzing the sentiment expressed in reviews, businesses can identify patterns that indicate potential fake or manipulated reviews. This allows them to maintain the integrity of their review systems and ensure that customers can make informed decisions based on genuine feedback.
Related Reading
• Sentiment Analysis Example
• Sentiment Analysis Social Media
• Brand Sentiment Analysis
• Online Sentiment Analysis
• How To Do Sentiment Analysis
• Sentiment Analysis Twitter
• Reddit Sentiment Analysis
• Sentiment Analysis Application
• Sentiment Analysis Of Reviews
• Lexicon Based Sentiment Analysis
• Sentiment Analysis Finance
• Sentiment Analysis Steps
• Amazon Review Sentiment Analysis
Rule-Based Sentiment Analysis

Sentiment analysis, also known as opinion mining, is a field of natural language processing that aims to determine the sentiment or emotional tone behind a piece of text. It has become increasingly important in today's digital age, where vast amounts of textual data are generated and shared online.
One of the approaches used in sentiment analysis is rule-based sentiment analysis. This method relies on a predefined set of rules or patterns to identify sentiments in text. Let's delve into how rule-based sentiment analysis works and explore its advantages and limitations.
Understanding Rule-Based Sentiment Analysis
Rule-based sentiment analysis involves creating a set of rules or patterns that map certain words or phrases to specific sentiments. These rules are typically crafted by linguists or domain experts who possess a deep understanding of the language and the specific domain being analyzed. The rules can be as simple as matching individual words to sentiments or as complex as using syntactic patterns to capture the sentiment.
To analyze a piece of text using rule-based sentiment analysis, the text is broken down into smaller units, such as sentences or phrases. Each unit is then compared against the predefined rules to determine the sentiment associated with it. The sentiment can be classified into categories such as positive, negative, or neutral, or it can be expressed as a score indicating the intensity of the sentiment.
Advantages of Rule-Based Sentiment Analysis
1. Linguistic Expertise
Rule-based sentiment analysis leverages the knowledge and expertise of linguists or domain experts who can fine-tune the rules to accurately capture sentiments. This human input ensures that the analysis aligns with the nuances and context of the language being analyzed.
2. Customizability
Rule-based sentiment analysis allows for customization, as the rules can be tailored to the specific requirements of a particular domain or use case. This flexibility enables the analysis to be more accurate and relevant to the specific context in which it is applied.
3. Interpretability
Rule-based sentiment analysis provides transparency in the analysis process. Since the rules are explicitly defined, it is easier to understand and interpret the results. This makes it useful for applications where explainability is crucial, such as in legal or regulatory contexts.
Limitations of Rule-Based Sentiment Analysis
1. Limited Coverage
Rule-based sentiment analysis heavily relies on predefined rules. If a sentiment is not captured by the existing rules, it may not be detected or appropriately classified. The analysis can be biased towards sentiments that are explicitly represented in the rules while overlooking more nuanced or emerging sentiments.
2. Scalability
Creating and maintaining a comprehensive set of rules for sentiment analysis can be a challenging and time-consuming task. As the volume and variety of text data increase, it becomes impractical to manually define rules for every possible sentiment or linguistic variation. This limits the scalability of rule-based sentiment analysis.
3. Context Sensitivity
Rule-based sentiment analysis may struggle with understanding the context in which a sentiment is expressed. The same words or phrases can have different meanings or sentiments depending on the surrounding context. Rules that are purely based on lexical matching may fail to capture these subtleties accurately.
4. Lack of Adaptability
Rule-based sentiment analysis is relatively static and lacks the ability to adapt to evolving language usage or changing sentiment patterns. As language evolves and new sentiments emerge, the predefined rules may become outdated and less effective in capturing the sentiment accurately.
Rule-based sentiment analysis offers a powerful approach to analyzing textual data and uncovering the sentiments behind them. It benefits from human expertise and customizability, making it a valuable tool in various domains. Its limitations, such as limited coverage, scalability challenges, context sensitivity, and lack of adaptability, highlight the need for alternative approaches to overcome these drawbacks and achieve more accurate and comprehensive sentiment analysis.
Aspect-Based Sentiment Analysis
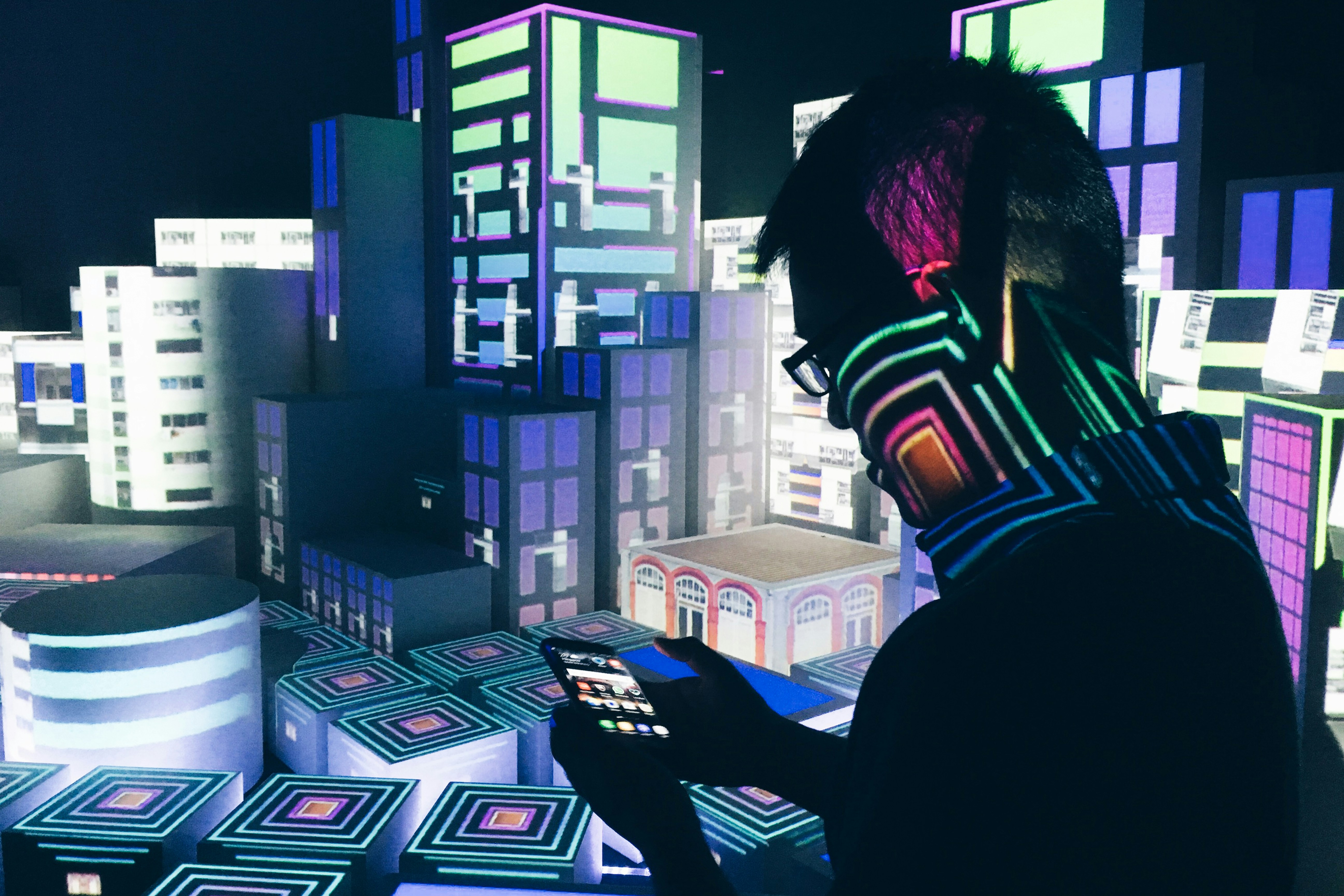
In the realm of sentiment analysis, one technique that has gained significant attention is aspect-based sentiment analysis. But what exactly is aspect-based sentiment analysis, and how can it provide more granular insights for businesses? Let's dive in and explore this fascinating area of sentiment analysis.
What is aspect-based sentiment analysis?
Aspect-based sentiment analysis is a methodology that goes beyond the overall sentiment of a piece of text and aims to identify and analyze the sentiment expressed towards specific aspects or features of a product, service, or experience. Instead of treating the entire text as a single entity, aspect-based sentiment analysis breaks it down into smaller units or aspects.
By identifying and analyzing these specific aspects, aspect-based sentiment analysis allows businesses to gain a more detailed understanding of customer opinions, preferences, and experiences. It helps to uncover hidden patterns and insights that might be missed when only considering the overall sentiment.
Enhancing granularity and accuracy
One of the key advantages of aspect-based sentiment analysis is its ability to provide more granular insights for businesses. By breaking down the text into specific aspects, allows companies to understand not only the overall sentiment but also the sentiment towards different elements of their products or services.
For example, let's consider a restaurant review. Traditional sentiment analysis might classify the review as positive or negative based on the overall sentiment expressed. Aspect-based sentiment analysis can identify and analyze the sentiment towards various aspects such as food quality, service, ambiance, and pricing. This detailed analysis enables businesses to identify areas of strength and weakness more precisely.
Aiding decision-making and strategy formulation
The granular insights provided by aspect-based sentiment analysis can greatly inform decision-making and strategy formulation for businesses. By understanding the sentiment associated with specific aspects, companies can prioritize areas for improvement, enhance customer satisfaction, and optimize their offerings.
For instance, a hotel chain using aspect-based sentiment analysis may discover that their customers consistently praise the cleanliness of their rooms but express dissatisfaction with the quality of the breakfast. Armed with this knowledge, the hotel chain can focus on improving their breakfast offerings, leading to increased customer satisfaction and loyalty.
Uncovering emerging trends and customer preferences
Another valuable application of aspect-based sentiment analysis lies in its ability to uncover emerging trends and customer preferences. By analyzing sentiment towards different aspects over time, businesses can identify shifting preferences and adapt their strategies accordingly.
For example, a fashion retailer using aspect-based sentiment analysis may discover that customers are increasingly expressing negative sentiments towards the sustainability practices of the industry. Armed with this insight, the retailer can take proactive measures to enhance their sustainability initiatives, thereby appealing to the evolving preferences of their target audience.
The power of aspect-based sentiment analysis
Aspect-based sentiment analysis empowers businesses to delve deeper into customer opinions and sentiments, enabling them to make more informed decisions, refine their strategies, and uncover emerging trends. By providing more granular insights, this methodology offers a robust framework for understanding the intricate nuances of customer sentiment, ultimately leading to enhanced customer satisfaction and business success.
Can Sentiment Analysis Detect Sarcasm?

Sentiment analysis, also known as opinion mining, is a powerful tool that allows us to understand and gauge the sentiment or emotion expressed in textual data. It has proven to be immensely valuable in applications such as brand monitoring, customer feedback analysis, and social media sentiment analysis. One question that often arises is whether sentiment analysis can accurately detect sarcasm and irony in textual data. We will explore this intriguing question and unravel the complexities of detecting sarcasm and irony through sentiment analysis.
Understanding Sarcasm: A Linguistic Art Form
Sarcasm is a form of verbal irony that expresses the opposite of what is actually meant. It relies on context, tone, and delivery to convey its intended meaning. Detecting sarcasm in written text can be challenging, as it lacks the vocal cues and facial expressions that are present in spoken language. Recent advancements in natural language processing and machine learning techniques have led to the development of sophisticated algorithms that can detect sarcasm in textual data.
The Role of Context and Semantic Analysis
Context is crucial in accurately detecting sarcasm in textual data. By considering the surrounding words, phrases, and sentences, sentiment analysis algorithms can gain a deeper understanding of the intended meaning behind a sarcastic statement. Semantic analysis, which focuses on the meaning of words and their relationships, plays a vital role in unraveling the layers of sarcasm. By analyzing the semantic incongruities in a sentence, sentiment analysis algorithms can identify instances of sarcasm with remarkable accuracy.
The Role of Sentiment Intensity and Contrast
Another important factor in detecting sarcasm through sentiment analysis is the intensity and contrast of sentiment. Sarcasm often involves a stark contrast between the words used and the underlying sentiment. For example, a sarcastic comment like "Oh, great job!" may contain positive words but carry a negative sentiment. By analyzing the sentiment intensity and contrasting sentiments within a sentence, sentiment analysis algorithms can discern the presence of sarcasm.
Navigating the Complexity of Irony
Irony, on the other hand, is a broader concept that encompasses various forms such as verbal irony, situational irony, and dramatic irony. It involves a discrepancy between what is expected and what actually occurs. Detecting irony in textual data can be even more challenging than sarcasm, as it requires a deep understanding of the cultural and situational context. While sentiment analysis algorithms have made significant strides in detecting irony, there is still room for improvement.
The Role of Pragmatics and World Knowledge
To accurately detect irony, sentiment analysis algorithms need to go beyond the literal meaning of words and delve into the realm of pragmatics. Pragmatics refers to the study of meaning in context, including the speaker's intentions, implied meanings, and shared knowledge between the speaker and the audience. By incorporating world knowledge and understanding the underlying nuances of a statement, sentiment analysis algorithms can uncover instances of irony that may be hidden to a literal interpretation.
Embracing the Challenge and Advancing Sentiment Analysis
While detecting sarcasm and irony through sentiment analysis presents challenges, researchers and data scientists are continuously pushing the boundaries of this field. By incorporating advanced techniques such as deep learning, sentiment analysis algorithms are evolving to better understand the complexities of language and the subtleties of human communication.
Sentiment analysis has the potential to accurately detect sarcasm and irony in textual data. By leveraging context, semantic analysis, sentiment intensity, contrast, pragmatics, and world knowledge, sentiment analysis algorithms can unravel the intricacies of these linguistic art forms. There is still much to be explored and refined in this field, and future advancements will undoubtedly enhance the accuracy and effectiveness of sentiment analysis in detecting sarcasm and irony.
Common Challenges With Sentiment Analysis & How To Overcome Them

Sentiment analysis, also known as opinion mining, is a powerful tool for businesses to understand customer feedback, gauge brand reputation, and make informed decisions. Like any data-driven process, sentiment analysis comes with its own set of challenges. We will explore the common challenges that businesses face in sentiment analysis and discuss strategies to overcome them.
1. Ambiguity in Sentiment Expression
One of the main challenges in sentiment analysis is dealing with the ambiguity of sentiment expression. Human language is complex, and sentiments can be expressed in various ways. For example, a positive sentiment may be conveyed through sarcasm, which can be difficult for a sentiment analysis model to detect. Similarly, negative sentiments may be disguised using euphemisms or subtle language.
To overcome this challenge, businesses can employ advanced natural language processing techniques. These techniques involve training sentiment analysis models on large datasets that include diverse language patterns and expressions. By exposing the model to a wide range of sentiments and their corresponding textual expressions, it becomes more adept at recognizing and interpreting nuanced sentiment expressions accurately.
2. Subjectivity and Contextual Analysis
Sentiments are highly subjective and can be heavily influenced by the context in which they are expressed. For instance, a customer review that praises the ambiance of a restaurant may not necessarily convey a positive sentiment towards the food or service. To accurately interpret sentiments, businesses need to consider the underlying context.
To address this challenge, businesses can incorporate contextual analysis into their sentiment analysis models. This involves analyzing the surrounding text or metadata to understand the broader context in which the sentiment is expressed. By considering factors such as the topic, entity, author's intent, and the sentiment's relevance to the overall document, businesses can obtain more accurate sentiment analysis results.
3. Handling Multilingual and Multicultural Data
Businesses often encounter multilingual and multicultural data when analyzing sentiments. Different languages and cultures have unique linguistic features and sentiment expressions, making sentiment analysis a complex task.
To overcome this challenge, businesses can leverage machine learning algorithms and techniques that are specifically designed for multilingual sentiment analysis. These algorithms can be trained on multilingual datasets to develop models that can accurately process sentiments in multiple languages. Businesses can collaborate with experts in different languages and cultures to gain insights into local sentiment expressions and ensure the accuracy of their sentiment analysis.
Potential Biases Associated with Sentiment Analysis
Sentiment analysis, like any data analysis process, is susceptible to biases. These biases can arise from various sources and can impact the accuracy and reliability of sentiment analysis results. Let's explore some of the potential biases associated with sentiment analysis:
1. Selection Bias
Selection bias occurs when the training data used to develop the sentiment analysis model is not representative of the target population. This can lead to skewed results, as the model is biased towards the sentiments expressed in the training data.
To mitigate selection bias, businesses should strive to collect diverse and representative training data. This can be achieved by incorporating data from different sources, regions, and demographics. Regularly updating the training data to reflect evolving sentiments in the target population is also crucial to reduce selection bias.
2. Cultural and Linguistic Bias
Sentiment analysis models can exhibit bias towards specific cultures or languages. This bias can arise due to the dominance of certain language or cultural patterns in the training data.
To address cultural and linguistic biases, businesses should ensure that their sentiment analysis models are trained on diverse datasets that include sentiments expressed across different cultures and languages. Businesses can employ post-processing techniques to identify and correct any bias present in the sentiment analysis results.
3. Labeling Bias
Labeling bias occurs when the human annotators who label the training data introduce their own biases into the sentiment analysis model. Annotators may have subjective interpretations or implicit biases that influence their labeling decisions.
To mitigate labeling bias, businesses should provide clear guidelines and instructions to the annotators, emphasizing the importance of objective and unbiased labeling. Regular quality checks and calibration sessions can also help identify and address any labeling biases that may arise.
Sentiment analysis is a valuable tool for businesses to gain insights into customer opinions and make data-driven decisions. It is important to be aware of the challenges and biases associated with sentiment analysis. By employing advanced natural language processing techniques, considering contextual analysis, and addressing potential biases, businesses can enhance the accuracy and reliability of their sentiment analysis results.
Step-by-Step Guide On How To Do Sentiment Analysis With Numerous
Watch this guide on how to use ChatGPT for classification and sentiment analysis in Google Sheets and Microsoft Excel with Numerous AI:
Google Sheets Guides
Microsoft Excel Guide
Measuring The ROI of Sentiment Analysis Initiatives

Tracking Customer Sentiment in Real-Time
Tracking customer sentiment in real-time has become crucial for businesses. By continuously monitoring and analyzing customer feedback, businesses can gain valuable insights into customer opinions, preferences, and satisfaction levels. This enables them to make data-driven decisions and take proactive measures to address any concerns or issues promptly.
Unlocking Insights
Real-time sentiment analysis involves using advanced technologies and algorithms to monitor customer sentiment across various platforms, such as social media, customer reviews, and online forums. By leveraging natural language processing and machine learning techniques, businesses can categorize customer sentiment into positive, negative, or neutral, and even identify specific emotions like joy, anger, or sadness.
By tracking customer sentiment in real-time, businesses can identify emerging trends, respond to customer complaints or negative feedback promptly, and capitalize on positive sentiments. This helps in building stronger customer relationships, improving brand reputation, and ultimately driving business growth.
Measuring the Effectiveness of Sentiment Analysis Initiatives
To measure the effectiveness of sentiment analysis initiatives, businesses can employ several key metrics and methodologies. These measures provide valuable insights into the impact of sentiment analysis on various aspects of the business, such as customer satisfaction, brand sentiment, and revenue generation. Here are a few ways businesses can measure the effectiveness of sentiment analysis initiatives:
1. Customer Satisfaction Scores
By comparing customer satisfaction scores before and after implementing sentiment analysis, businesses can assess the impact on customer happiness levels. This can include metrics like Net Promoter Score (NPS) or customer satisfaction surveys.
2. Brand Sentiment Analysis
Analyzing changes in brand sentiment over time can help businesses understand the impact of sentiment analysis initiatives on brand perception. This can be done by monitoring online mentions, social media sentiment, or conducting brand sentiment surveys.
3. Customer Retention Rates
By measuring the retention rates of customers who have had their sentiment analyzed, businesses can evaluate the impact of sentiment analysis on customer loyalty and retention. Higher retention rates indicate that sentiment analysis initiatives are successfully addressing customer concerns and improving satisfaction levels.
4. Revenue Generation
Businesses can analyze the correlation between sentiment analysis insights and revenue generation. By tracking the sentiment of customers who made purchases or engaged in positive brand interactions, businesses can determine the impact sentiment analysis has on driving revenue.
5. Resolution Time
By tracking the time taken to resolve customer complaints or issues flagged through sentiment analysis, businesses can assess the effectiveness of their response systems. A decrease in resolution time indicates that sentiment analysis is enabling businesses to address customer concerns more efficiently.
Measuring the return on investment (ROI) of sentiment analysis initiatives is crucial for businesses to evaluate the value and effectiveness of their efforts. By tracking and analyzing these metrics and methodologies, businesses can make data-driven decisions to optimize their sentiment analysis strategies and drive positive outcomes.
Key Success Factors When Implementing Sentiment Analysis To Make More Money
One of the key success factors for implementing sentiment analysis in a business setting is the use of high-quality data. Sentiment analysis relies on accurate and relevant data to provide meaningful insights. It is essential to collect data from diverse and representative sources, such as customer reviews, social media posts, and surveys. The larger the dataset, the more comprehensive and reliable the sentiment analysis results will be. Data should be regularly updated to capture the dynamic nature of sentiment. By ensuring the use of high-quality data, businesses can make informed decisions based on accurate sentiment analysis.
The Significance of Text Preprocessing in Sentiment Analysis
Text preprocessing is a crucial step in sentiment analysis that involves cleaning and preparing textual data for analysis. It plays a vital role in enhancing the accuracy and efficiency of sentiment analysis algorithms. Text preprocessing techniques include removing punctuation, stop words, and special characters, as well as stemming or lemmatizing words to reduce their variations.
By removing noise and irrelevant information, text preprocessing helps to focus on the sentiment-bearing words and phrases, improving the overall accuracy of sentiment analysis. Thus, businesses should prioritize text preprocessing to ensure the reliability of their sentiment analysis results.
The Role of Machine Learning Algorithms in Sentiment Analysis
Machine learning algorithms play a fundamental role in sentiment analysis. These algorithms use statistical techniques to learn from data patterns and make predictions about sentiment. Supervised learning algorithms, such as Support Vector Machines (SVM) and Naive Bayes, are commonly used in sentiment analysis to classify text into positive, negative, or neutral sentiments.
These algorithms learn from labeled training data, enabling them to accurately classify unseen text. Unsupervised learning algorithms, such as clustering algorithms, are also utilized to identify patterns and group similar sentiments together. By leveraging machine learning algorithms, businesses can automate sentiment analysis, saving time and resources while ensuring accurate results.
The Importance of Domain Adaptation in Sentiment Analysis
Domain adaptation refers to the process of adapting sentiment analysis models to specific business domains. Sentiment analysis models trained on general datasets may not perform well in industry-specific contexts due to differences in language, vocabulary, and sentiment expressions.
By fine-tuning or retraining models using domain-specific data, businesses can improve the accuracy and relevance of sentiment analysis results. Domain adaptation techniques include transfer learning, where models leverage knowledge learned from one domain to another, and domain-specific feature engineering, where sentiment features specific to the domain are extracted. Implementing domain adaptation ensures that sentiment analysis is tailored to the unique needs and characteristics of a business, leading to more meaningful insights.
The Benefits of Real-time Sentiment Analysis for Business Decisions
Real-time sentiment analysis enables businesses to respond swiftly to customer sentiment and make timely decisions. By monitoring sentiment in real-time, businesses can identify emerging trends, track customer satisfaction levels, and detect potential issues early on. Real-time sentiment analysis also enables businesses to adapt their marketing strategies, improve customer service, and enhance brand reputation.
With the rapid pace of social media and online communication, real-time sentiment analysis is crucial for staying ahead of the competition and maintaining a strong presence in the market. By leveraging real-time sentiment analysis, businesses can make proactive decisions that positively impact their bottom line.
The Role of Human Validation in Sentiment Analysis
While sentiment analysis algorithms are powerful tools, they are not infallible. Human validation is essential to ensure the accuracy and reliability of sentiment analysis results. Humans can provide context and interpret sentiment nuances that algorithms may not capture. Human validation can be done through manual annotation or by using crowdsourcing platforms to validate sentiment labels.
By combining the strengths of machine learning algorithms and human judgment, businesses can achieve higher accuracy in sentiment analysis and gain deeper insights into customer sentiment. Human validation serves as a quality control measure, ensuring that sentiment analysis is truly reflective of the customers' emotions and opinions.
The Impact of Sentiment Analysis Integration on Business Success
Integrating sentiment analysis into business processes and decision-making has a significant impact on overall business success. By understanding customer sentiment, businesses can tailor their products, services, and marketing efforts to meet customer needs and preferences. Sentiment analysis can also help identify and resolve customer issues, leading to improved customer satisfaction and loyalty.
Sentiment analysis enables businesses to track their brand reputation and sentiment trends over time, allowing them to make data-driven decisions. By leveraging sentiment analysis insights, businesses can gain a competitive edge, optimize their strategies, and achieve long-term success in the market.
How Sentiment Analysis Can Be Combined With Other Analytics Techniques

Sentiment analysis and social network analysis are both powerful analytics techniques that can provide valuable insights into customer behavior and preferences. By combining these two techniques, businesses can gain a deeper understanding of the relationships and sentiments within their social networks, allowing them to make more informed decisions and improve their marketing strategies.
Unlocking Insights
Social network analysis is the study of social relationships and interactions between individuals or groups. It involves analyzing the structure and dynamics of social networks, including the relationships between individuals, the flow of information, and the influence of key actors. By looking at patterns of connections and interactions, social network analysis can reveal valuable insights about customer behavior and preferences.
Decoding Emotions
Sentiment analysis, on the other hand, focuses on extracting and analyzing subjective information from text data. It involves determining the sentiment or emotion expressed in a piece of text, such as a social media post or customer review. Sentiment analysis can help businesses understand how customers feel about their products or services, identify emerging trends and issues, and measure customer satisfaction.
Comprehensive Understanding
By combining sentiment analysis with social network analysis, businesses can gain a more comprehensive understanding of their social networks. They can analyze the sentiment of individual nodes (such as customers or influencers) within the network and identify patterns of sentiment within different groups or communities. This can provide insights into the overall sentiment towards the business, specific products or services, or even specific individuals within the network.
Influencer Dynamics
For example, a company could use sentiment analysis to analyze the sentiment of customer reviews on social media platforms. By mapping the relationships between customers and influencers within their social network, they can identify key influencers who have a significant impact on the sentiments of others. This can help them determine which influencers to target for marketing campaigns or how to address negative sentiment within the network.
Community Connection
Sentiment analysis can be used to identify sentiment clusters within a social network. By grouping customers with similar sentiments together, businesses can identify communities with shared preferences or concerns. This can help them tailor their marketing messages or develop targeted campaigns for specific groups within their social network.
Using Sentiment Analysis for Customer Segmentation

Customer segmentation is the process of dividing a customer base into distinct groups based on shared characteristics or behaviors. It helps businesses better understand their customers, target their marketing efforts, and tailor their products or services to meet specific needs. By incorporating sentiment analysis into the customer segmentation process, businesses can gain a deeper understanding of customer preferences and emotions, allowing for more effective segmentation strategies.
Emotional Insights
Sentiment analysis can provide valuable insights into the emotional drivers behind customer behavior. By analyzing the sentiment expressed in customer feedback, reviews, or social media posts, businesses can identify the emotions that impact customer decision-making. This information can be used to segment customers based on their emotional needs or preferences.
Applying Sentiment Analysis to Hotel Reviews
For example, a hotel chain could use sentiment analysis to segment its customers based on their experiences and emotions expressed in online reviews. They could identify customers who have had positive experiences and are likely to become loyal advocates. They could also identify customers who have had negative experiences and address their concerns in order to improve their satisfaction and loyalty.
Behavioral Dynamics
In addition to emotional segmentation, sentiment analysis can also be used for behavioral segmentation. By analyzing the sentiment expressed in customer interactions or feedback, businesses can identify different customer segments based on their purchasing behaviors or engagement levels. This information can help businesses target specific customer segments with personalized marketing messages or offers.
Targeting Customer Segments
For instance, an e-commerce company could use sentiment analysis to segment their customers based on their level of engagement and satisfaction with the brand. They could identify highly satisfied customers who are likely to make repeat purchases and target them with loyalty rewards or exclusive offers. They could also identify dissatisfied customers who are at risk of churning and develop targeted retention strategies to prevent them from leaving.
Holistic Approach
Sentiment analysis can be a powerful tool when combined with other analytics techniques, such as social network analysis or customer segmentation. By incorporating sentiment analysis into these techniques, businesses can gain deeper insights into customer preferences, emotions, and relationships. This can lead to more effective marketing strategies, improved customer satisfaction, and ultimately, increased business success.
Make Decisions At Scale Through AI In Spreadsheets With Numerous
Harnessing the potential of AI technology, Numerous.ai offers a range of innovative solutions for content marketers, ecommerce businesses, and more. By seamlessly integrating with popular tools like Microsoft Excel and Google Sheets, Numerous.ai empowers users to accomplish a multitude of tasks effortlessly. Let's take a closer look at the remarkable capabilities of Numerous.ai and how it can revolutionize your business.
Sentiment Analysis and Product Categorization
Understanding customer sentiment is crucial for any business. Numerous.ai employs sentiment analysis algorithms to categorize products based on customer feedback, allowing you to gauge customer satisfaction levels and identify areas for improvement. This insightful data empowers you to make data-driven decisions that drive growth.
Spreadsheet Function Automation
Streamline your workflow by automating spreadsheet functions with Numerous.ai. Whether you need to perform simple calculations or complex data manipulations, Numerous.ai delivers lightning-fast results. By automating repetitive tasks, you can focus on strategic decision-making and drive productivity.
Business Decisions at Scale
Leveraging AI-powered insights, Numerous.ai enables you to make informed business decisions at scale. By synthesizing vast amounts of data and providing actionable recommendations, Numerous.ai equips you with the tools to navigate complex market landscapes and gain a competitive edge.
Embrace the Power of Numerous.ai Today
With its versatile applications, Numerous.ai empowers businesses to unlock the full potential of AI technology. Whether you're a content marketer seeking to optimize productivity or an ecommerce business aiming to enhance customer experience, Numerous.ai offers a comprehensive suite of AI-powered solutions. Start harnessing the power of Numerous.ai today and propel your business to new heights.
Related Reading
• Sentiment Analysis Api
• Sentiment Analysis Software
• Ai Sentiment Analysis
• Sentiment Analysis In Excel
• Online Sentiment Analysis Tool
• Gpt3 Sentiment Analysis
• Sentiment Analysis Google Sheets